The innovative Diffusion2 framework merges video and multi-view models to forge dynamic 3D content, sidestepping the need for extensive 4D data.
- Innovative 4D Generation: Diffusion2 introduces a groundbreaking approach to creating dynamic 3D content, utilizing a novel denoising strategy that combines video and multi-view diffusion models.
- High-Efficiency Content Creation: The framework boasts remarkable efficiency in generating dense, synchronized multi-view videos, significantly reducing the time required to produce 4D content.
- Adaptability to Various Prompts: Demonstrating versatility, Diffusion2 shows a strong ability to adapt to a wide range of prompts, highlighting its potential for diverse applications in 4D content generation.
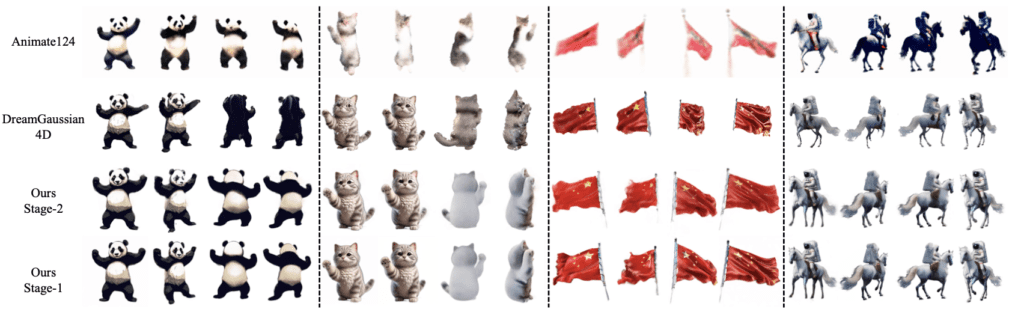
The realm of 3D content generation stands on the cusp of a transformative breakthrough with the advent of Diffusion2, a pioneering framework designed to tackle the challenges of dynamic 3D, or 4D, content creation. This novel approach is poised to reshape how creators approach the production of multi-dimensional content, offering a path forward that circumvents the traditional barriers associated with 4D data scarcity.
Bridging the Gap in 4D Content Generation
Traditionally, the generation of 4D content has been hindered by the lack of synchronized multi-view video data, making direct adaptation of existing 3D-aware image diffusion models impractical. Diffusion2 addresses this challenge head-on by effectively leveraging the available video and 3D data to train specialized diffusion models that provide the necessary dynamic and geometric priors for 4D content creation.
A Synergistic Denoising Strategy
At the heart of Diffusion2’s innovation is a strategic denoising process that synergizes video and multi-view diffusion models. This process is predicated on the understanding that elements within the multi-view, multi-frame image arrays are conditionally independent given certain parameters, a principle that facilitates the direct sampling of synchronized multi-view videos. This denoising strategy not only enhances the geometric consistency and temporal smoothness of the generated content but also ensures high parallelism in image generation, significantly boosting efficiency.
Efficient and Flexible 4D Reconstruction
The framework’s ability to quickly generate dense image arrays that are then fed into a modern 4D reconstruction pipeline underscores its efficiency, with the entire process of creating 4D content taking just a few minutes. Moreover, Diffusion2’s flexibility in adapting to various prompts ensures its applicability across a broad spectrum of 4D content generation tasks, from entertainment and media to scientific visualization and beyond.
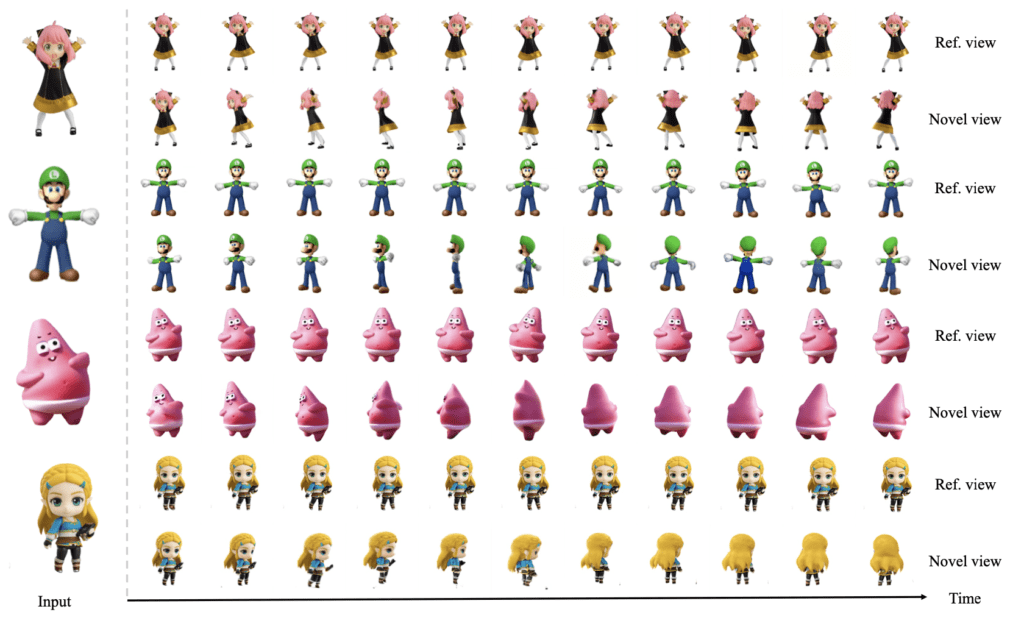
Implications for Future Research and Applications
The implications of Diffusion2’s capabilities extend far beyond immediate practical applications. By demonstrating that dynamic 3D content can be generated without direct reliance on extensive 4D datasets, Diffusion2 opens new avenues for leveraging the scalability of foundational video and multi-view diffusion models. This approach not only makes 4D content creation more accessible but also sets a new benchmark for future research in the field, encouraging further exploration into the combination of geometrical and dynamic priors.
Diffusion2 represents a significant leap forward in the generation of dynamic 3D content. By harmonizing the strengths of video and multi-view diffusion models within a highly efficient framework, it offers a novel solution to the longstanding challenges of 4D content creation, paving the way for innovative applications and further advancements in the domain of multi-dimensional content generation.
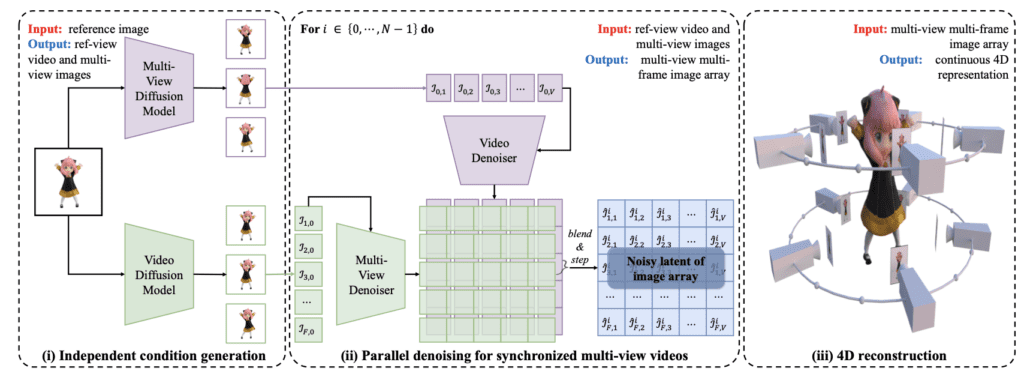