New Study Reveals Optimized Design Strategies for Enhanced Visual Perception in Multimodal Models.
- Streamlined Design Approach: The study shows that concatenating visual tokens from multiple vision encoders can be as effective as more complex integration methods, simplifying the design of multimodal models without sacrificing performance.
- Introduction of Pre-Alignment: A novel technique called Pre-Alignment bridges the gap between vision encoders and language tokens, significantly improving model coherence and accuracy in interpreting visual information.
- EAGLE Model Excellence: The new family of MLLMs, named EAGLE, surpasses existing open-source models on key benchmarks, demonstrating the efficacy of systematic design choices and optimized fusion methods in enhancing multimodal capabilities.
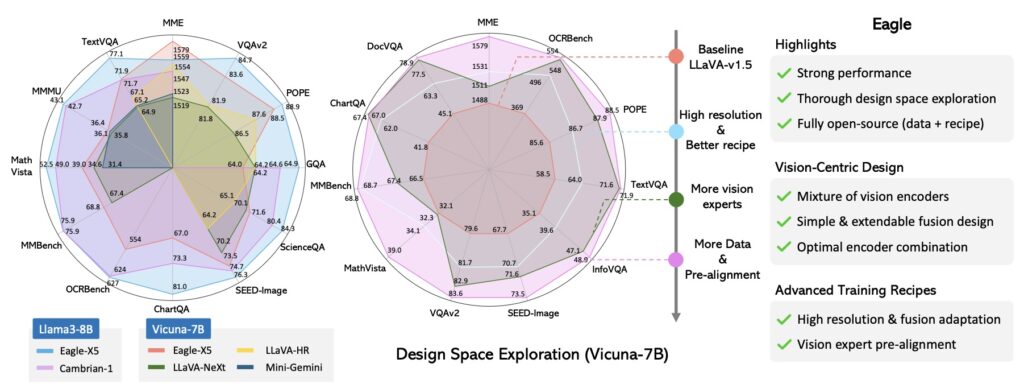
In the evolving landscape of artificial intelligence, the ability of multimodal large language models (MLLMs) to interpret and integrate complex visual information is increasingly critical. A new study, exploring the design space for MLLMs, introduces EAGLE—a family of models that exemplifies the benefits of a well-structured mixture of vision encoders.
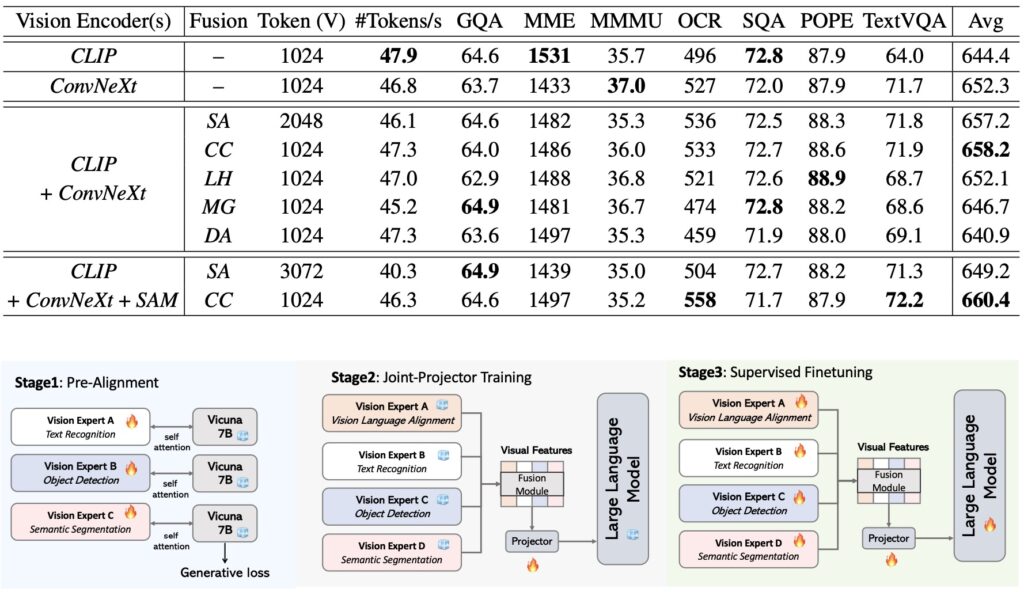
Simplifying the Design
The research from Nvidia highlights that the effectiveness of multimodal models can be achieved through simpler methods than previously thought. By concatenating visual tokens from different vision encoders, the study reveals that this straightforward approach can rival more intricate mixing strategies. This finding suggests that simplicity in design does not compromise effectiveness, providing a more streamlined approach to model development.
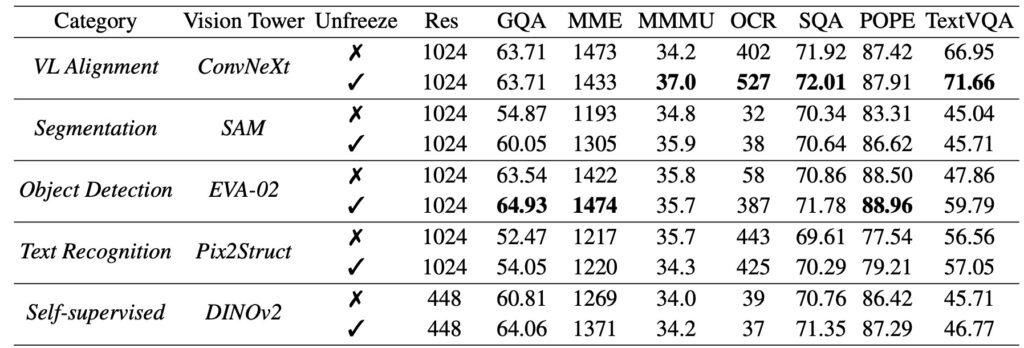
Pre-Alignment: Bridging the Gap
A key innovation introduced in the study is the Pre-Alignment technique. This method enhances the coherence between vision encoders and language tokens, improving the model’s ability to integrate and interpret visual information in context. By aligning these components more effectively, Pre-Alignment contributes to reducing errors and enhancing overall performance.
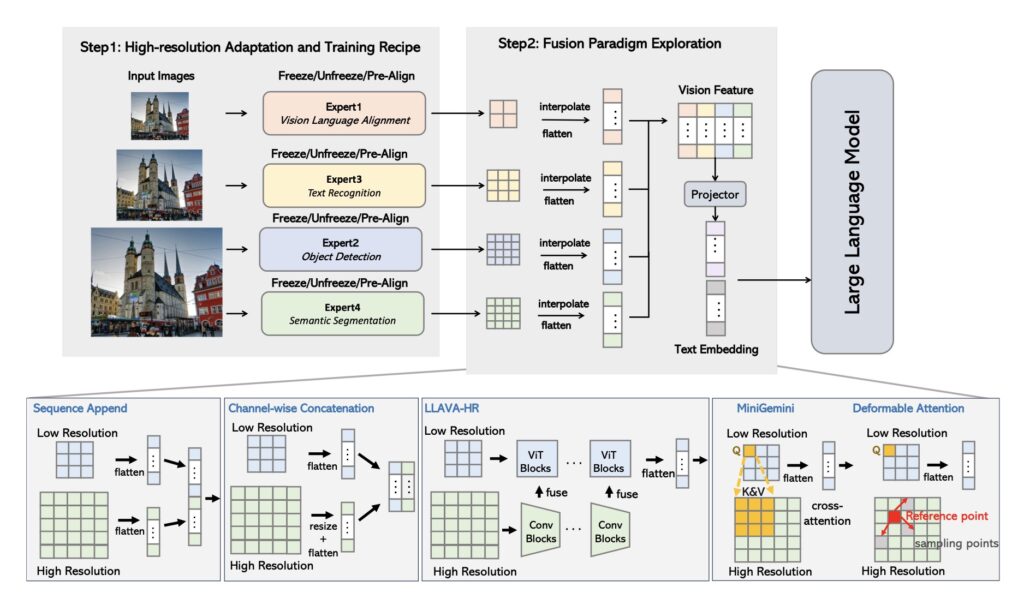
The EAGLE Advantage
The culmination of these advancements is the EAGLE model family, which has outperformed other leading open-source MLLMs on major benchmarks. This success underscores the importance of systematic design choices and optimized fusion methods in creating high-performance multimodal models. The study’s approach provides a new foundation for designing vision encoders in MLLMs, promising to inspire future innovations in the field.
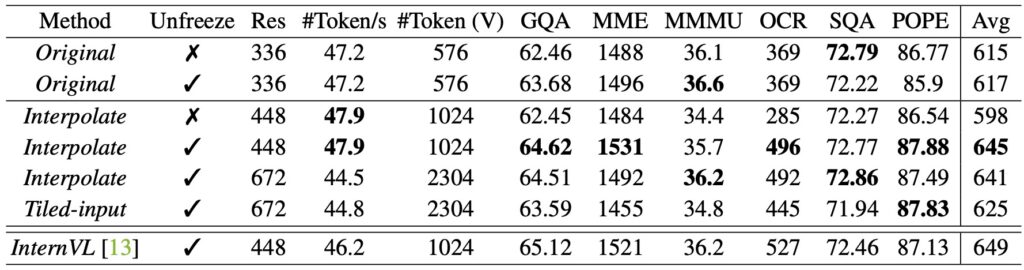
The EAGLE study offers valuable insights into designing effective multimodal models, demonstrating that optimizing basic design elements and incorporating novel techniques like Pre-Alignment can lead to significant improvements in performance and coherence.