How MetaAI’s COMPositional Atomic-to-Complex Visual Capability Tuning Redefines Multimodal Learning
- COMPACT, developed by MetaAI, introduces a novel training method for Multimodal Large Language Models (MLLMs) by focusing on compositional complexity, enabling models to tackle intricate vision-language tasks with remarkable efficiency.
- By generating a balanced dataset that combines atomic visual capabilities into composite skills, COMPACT achieves performance comparable to the full-scale LLaVA-665K Visual Instruction Tuning (VIT) while using less than 10% of the data budget.
- Despite its groundbreaking results, COMPACT faces challenges such as reliance on closed-source models for data generation and limitations in addressing knowledge-intensive tasks, with future work aimed at overcoming these hurdles.
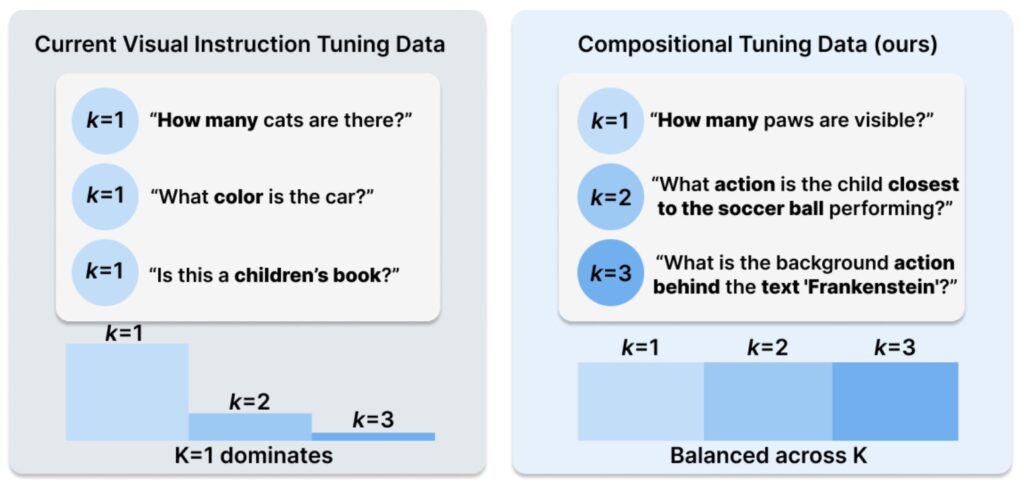
The world of artificial intelligence is witnessing a transformative shift with the introduction of COMPACT (COMPositional Atomic-to-Complex Visual Capability Tuning) by MetaAI. Multimodal Large Language Models (MLLMs) have long excelled at straightforward vision-language tasks, such as identifying objects in an image or answering basic questions about visual content. However, when faced with complex challenges that require multiple capabilities—like recognizing objects, counting them, and understanding their spatial relationships—these models often falter. The root of this struggle lies in the traditional approach to Visual Instruction Tuning (VIT), which prioritizes scaling data volume over the compositional complexity of training examples. COMPACT seeks to change this paradigm by offering a data-efficient, structured approach to training that empowers MLLMs to master intricate tasks through compositional generalization.
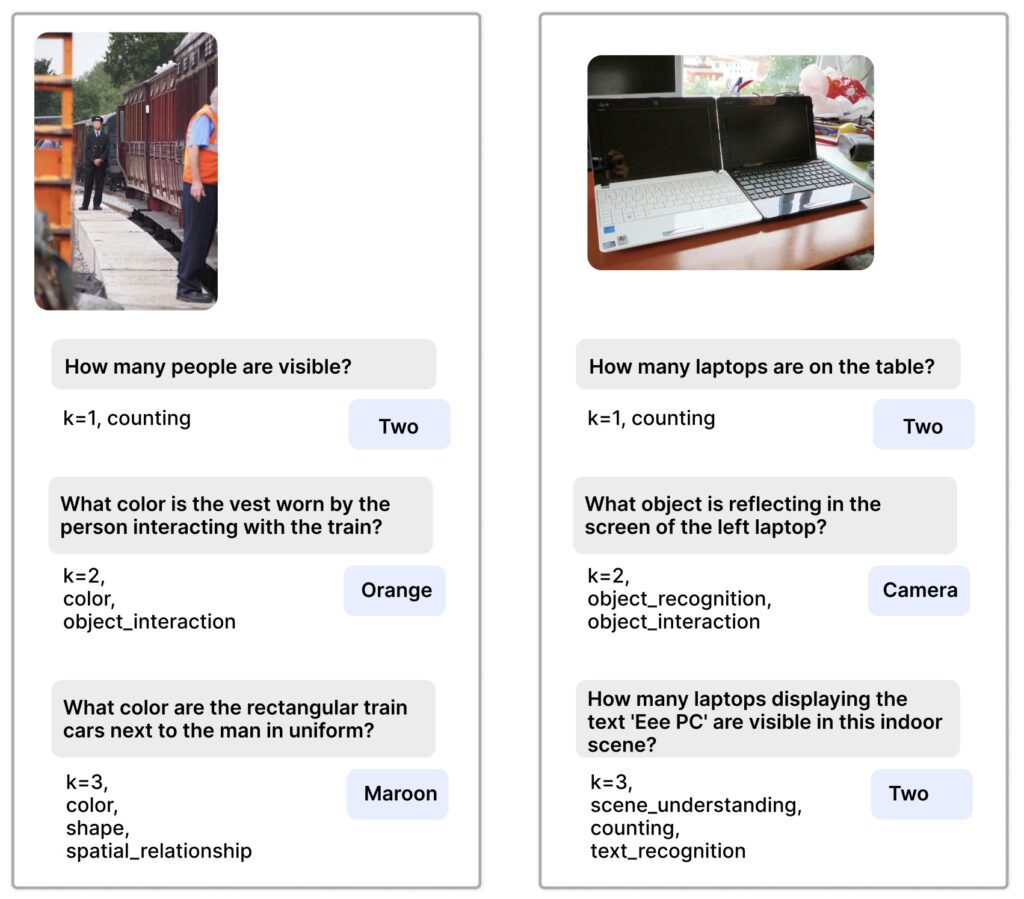
At its core, COMPACT redefines how MLLMs learn by generating a training dataset that explicitly controls for compositional complexity. This means breaking down complex tasks into atomic visual capabilities—foundational skills such as object recognition, spatial reasoning, and shape attribution—and systematically combining them into composite capabilities. For instance, to determine how objects of different colors are spatially oriented, a model must integrate skills like object identification, color attribution, and spatial understanding. COMPACT defines the number of atomic capabilities required for a task as its compositional complexity, denoted as ‘k,’ and generates training data that scales from simple (k=1) to more intricate levels (k=2 or 3). With just 32,000 samples of compositional tuning data and a mere 5% of the LLaVA-665K VIT dataset, COMPACT matches the performance of the full-scale VIT, achieving a relative score of 100.18%. Even more impressively, it demonstrates exceptional generalization on complex tasks, outperforming traditional methods on benchmarks like MMStar with an 83.3% improvement and MM-Vet with a 94.0% boost, particularly on questions requiring four or more atomic capabilities.
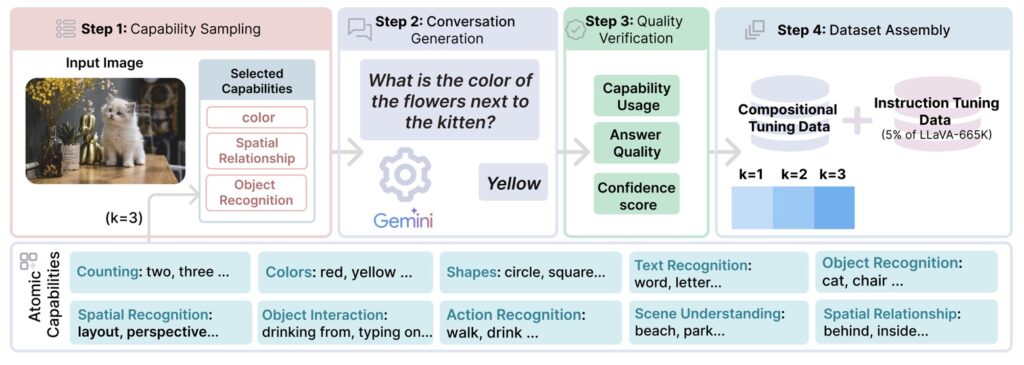
What sets COMPACT apart is its balanced distribution of compositional complexity within the training data. Unlike the LLaVA-665K VIT dataset, which exhibits a “complexity cliff” with a scarcity of higher-k samples, COMPACT ensures a fair representation of tasks across varying levels of difficulty. This balance is critical to its success, as evidenced by a comparative analysis: when COMPACT’s dataset was intentionally unbalanced to mimic the distribution of LLaVA-665K, its performance dropped to 96.28%, close to a random baseline. However, with its original balanced design, performance soared to 98.83%, underscoring that the gains stem directly from the equitable inclusion of complex examples. This structured approach not only enhances efficiency—using less than 10% of the data budget of full-scale VIT—but also enables models to generalize to multi-capability tasks without needing explicit decomposition during inference.
Despite its remarkable achievements, COMPACT is not without limitations. One significant challenge is its reliance on closed-source models like Gemini for data generation, which may introduce biases or compositional limitations into the dataset. Additionally, the process of creating this data is costly, potentially hindering reproducibility for other researchers. To mitigate this, MetaAI plans to publicly release the generated data, fostering collaboration and further exploration. Another constraint is COMPACT’s focus on vision-centric capabilities, which means it may not be ideally suited for knowledge-intensive tasks outside the realm of visual reasoning. Detailed discussions on these results are available in the project’s appendix, highlighting the need for tailored approaches in such domains.
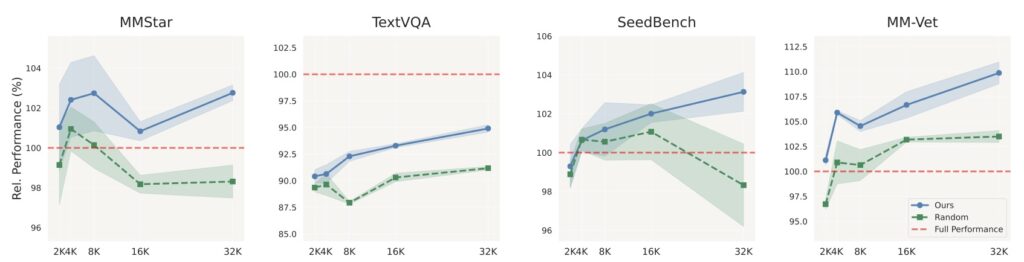
Looking ahead, the potential for COMPACT to evolve is immense. Currently, the dataset is limited to compositional complexities up to k=3 due to the decreasing reliability of closed-source models at higher levels, where integration of atomic capabilities becomes inconsistent or erroneous. Future work aims to address this by exploring hierarchical composition methods or hybrid data generation pipelines that incorporate multiple sources and verification steps. Additionally, integrating explicit reasoning techniques, such as step-by-step task decomposition, could further enhance the model’s ability to handle complex challenges while maintaining data efficiency. The vision is to extend COMPACT to accommodate higher-order complexities (k>3), paving the way for MLLMs that can tackle even more sophisticated multimodal tasks.
COMPACT represents a paradigm shift in how we approach multimodal learning. It challenges the conventional wisdom of “more data, better results” by demonstrating that smarter, structured data can yield comparable or superior outcomes with significantly fewer resources. This data-efficient pathway not only reduces computational costs but also opens doors for smaller research teams or organizations with limited budgets to contribute to cutting-edge AI development. Moreover, COMPACT’s emphasis on compositional generalization mirrors how humans learn—building complex understanding from simpler building blocks—offering a glimpse into how AI might one day emulate human-like reasoning in visual and linguistic contexts.
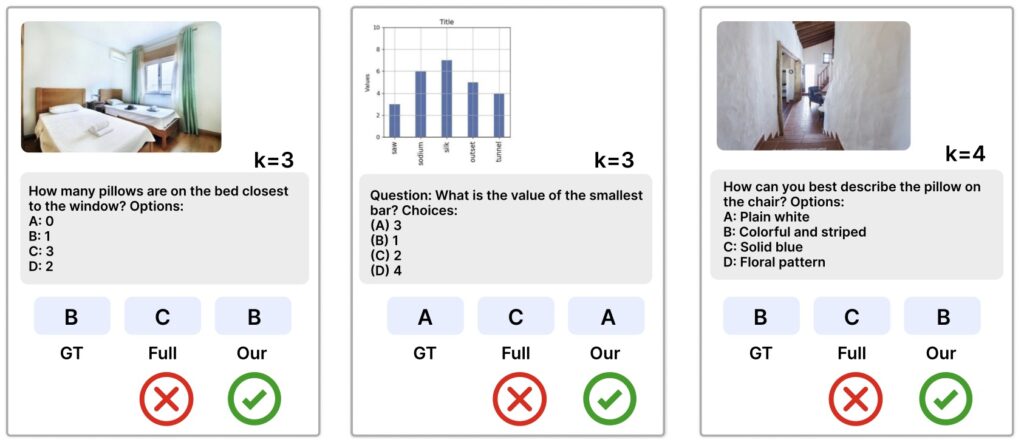
COMPACT from MetaAI is a groundbreaking step forward for Multimodal Large Language Models, proving that compositional complexity is key to unlocking their full potential. By systematically combining atomic visual capabilities into composite skills, it achieves unparalleled efficiency and performance on complex tasks. While challenges remain, particularly in data generation and scope, the future of COMPACT holds promise for even greater advancements. As AI continues to evolve, approaches like COMPACT will likely serve as cornerstones for building models that can navigate the intricate interplay of vision and language with human-like finesse.