A groundbreaking mathematical method demystifies how neural networks make decisions, paving the way for more trustworthy AI systems.
- Breaking the AI Black Box: Researchers at Western University have developed a mathematical framework that explains how neural networks process data.
- Real-World Applications: The technique was tested on image segmentation tasks and could be applied to various AI domains, from secure messaging to hybrid systems connecting artificial and biological neural networks.
- Future of Explainable AI: This method provides insights into AI decision-making, fostering trust and transparency in advanced machine learning systems.
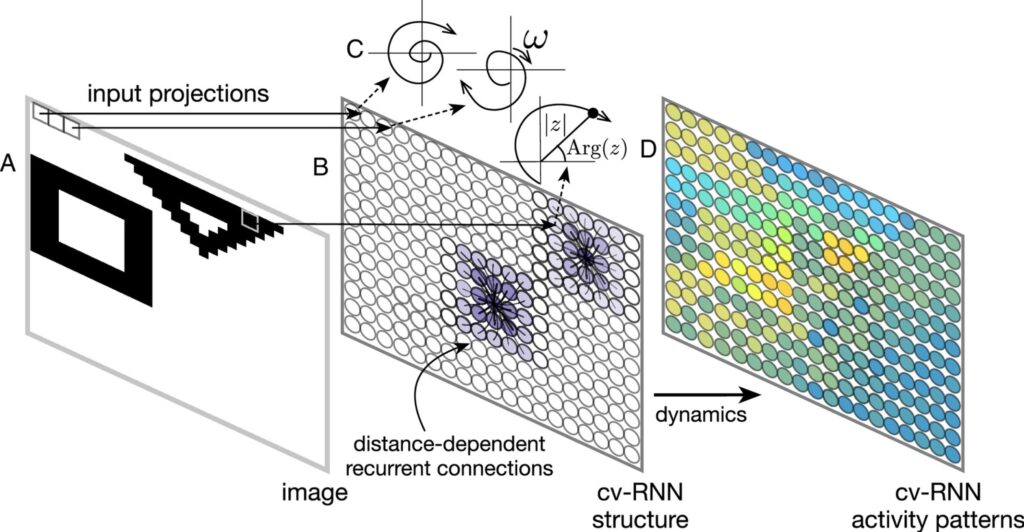
Neural networks, the backbone of many AI systems, are often called “black boxes” due to the difficulty in understanding how they process data. A team of researchers at Western University, led by mathematics professor Lyle Muller, has developed a groundbreaking mathematical approach that sheds light on this enigmatic process. Their method enables scientists to “open the black box” and precisely map how neural networks make decisions.
Published in PNAS and involving international collaboration, the research promises to make AI systems more explainable and trustworthy, which is critical for deploying these technologies in sensitive areas like medicine and autonomous systems.
The Power of Math in Image Segmentation
The research team first applied their framework to image segmentation, a core task in computer vision where neural networks divide images into distinct parts, such as separating objects from backgrounds. Starting with simple shapes, the team created a neural network capable of analyzing images. By solving equations that governed the network’s activity, they could explain every computational step the network took.
The results were surprising: their approach not only worked on basic images but also extended to natural images like photographs of polar bears and birds. This flexibility highlights the potential of mathematically explainable networks to handle diverse and complex tasks.
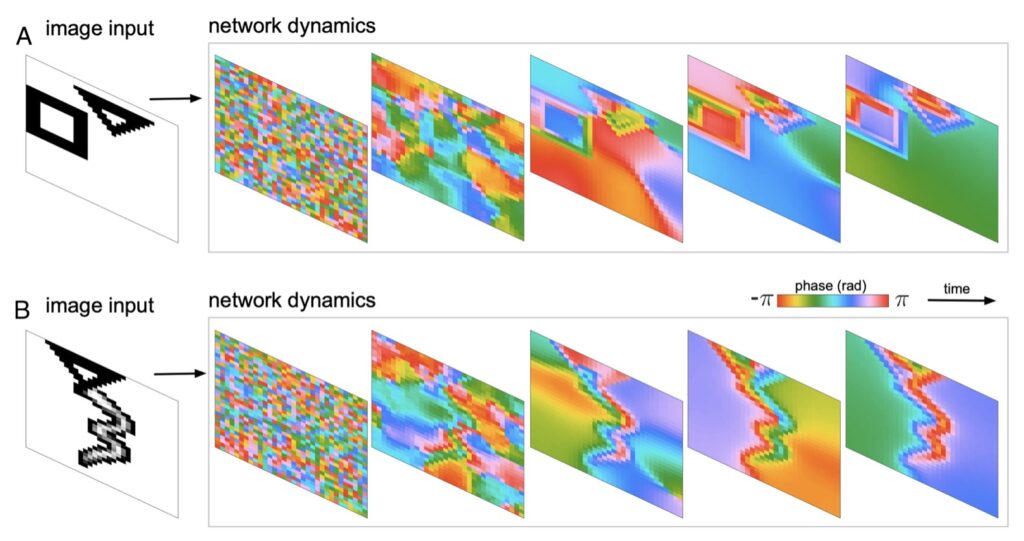
Broader Applications of Explainable AI
The implications of this research go beyond image segmentation. In a related study, the team developed a similar framework for tasks such as secure message-passing, basic logic operations, and memory functions. They even collaborated with the Schulich School of Medicine & Dentistry to connect their network to living brain cells, creating a hybrid system that bridges artificial and biological neural networks.
“This kind of fundamental understanding is crucial as we continue to develop more sophisticated AI systems that we can trust and rely on,” said Roberto Budzinski, a post-doctoral scholar on the project.
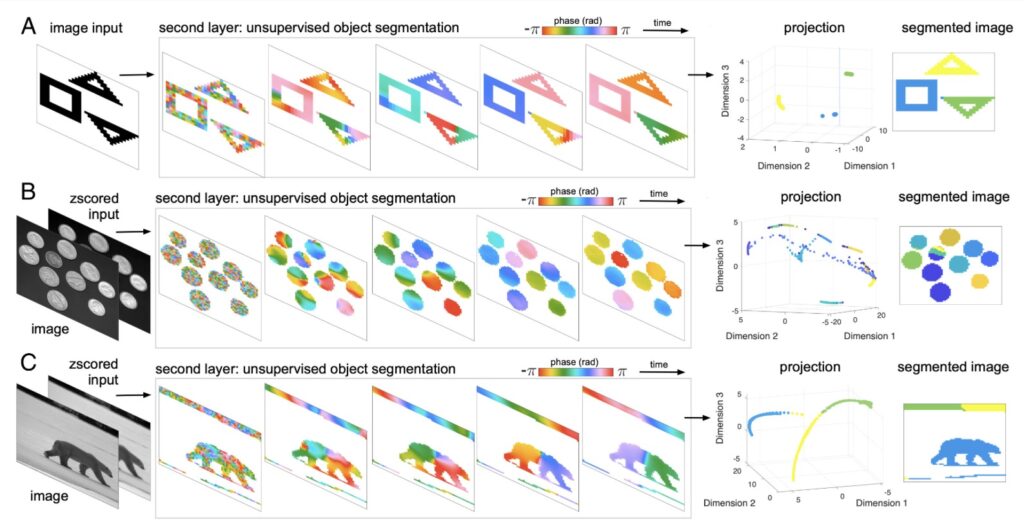
A New Era of Explainable AI
This research represents a significant step forward in explainable AI, a field dedicated to understanding and interpreting how AI systems make decisions. The mathematical framework developed by the Western team offers not just theoretical insights but practical tools for advancing AI transparency.
As AI continues to influence areas like healthcare, transportation, and finance, understanding how these systems work is critical for ensuring their safety, reliability, and ethical use.
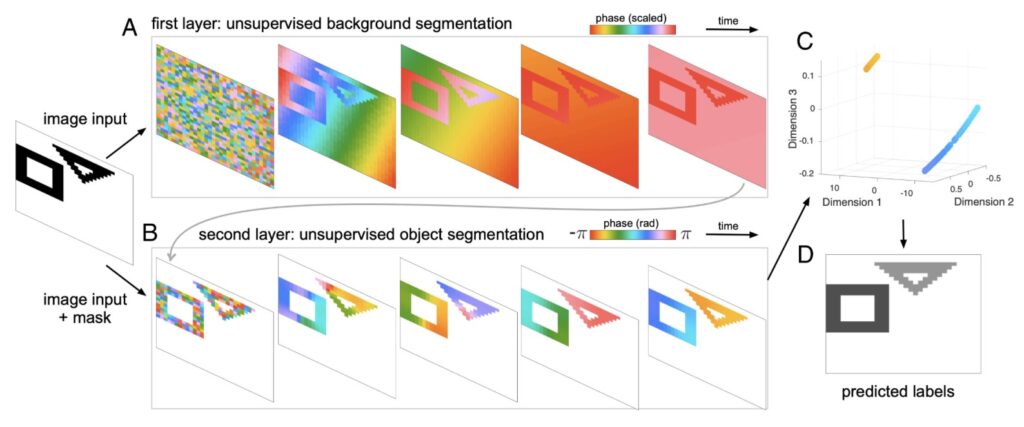
The Road Ahead
The ability to mathematically explain neural networks’ decision-making processes has opened new pathways in AI research and development. By demystifying how these systems operate, researchers can build more transparent and robust AI solutions, paving the way for their safe integration into real-world applications.
Western University’s innovation in this space is a testament to the transformative potential of interdisciplinary research, combining mathematics, neuroscience, and machine learning to unlock AI’s full potential.