GoodAI Advances AI with New Features and Dynamic Tests in Latest LTM Benchmark Release
- Continuous Benchmark Development: The GoodAI LTM Benchmark is continuously evolving, introducing new tasks and features to push the capabilities of conversational AI agents further, especially in long-term memory.
- Integration of Dynamic Testing: Moving away from static tests, the new dynamic tests allow for more natural interactions and the ability to adjust the conversation based on the AI’s responses, enhancing the realism of testing scenarios.
- Introduction of Percentage Waits: This innovative feature enables more flexible and realistic timing in tests, allowing tasks to be paused until a certain percentage of other tasks are completed, thus improving the flow and timing of interactions.
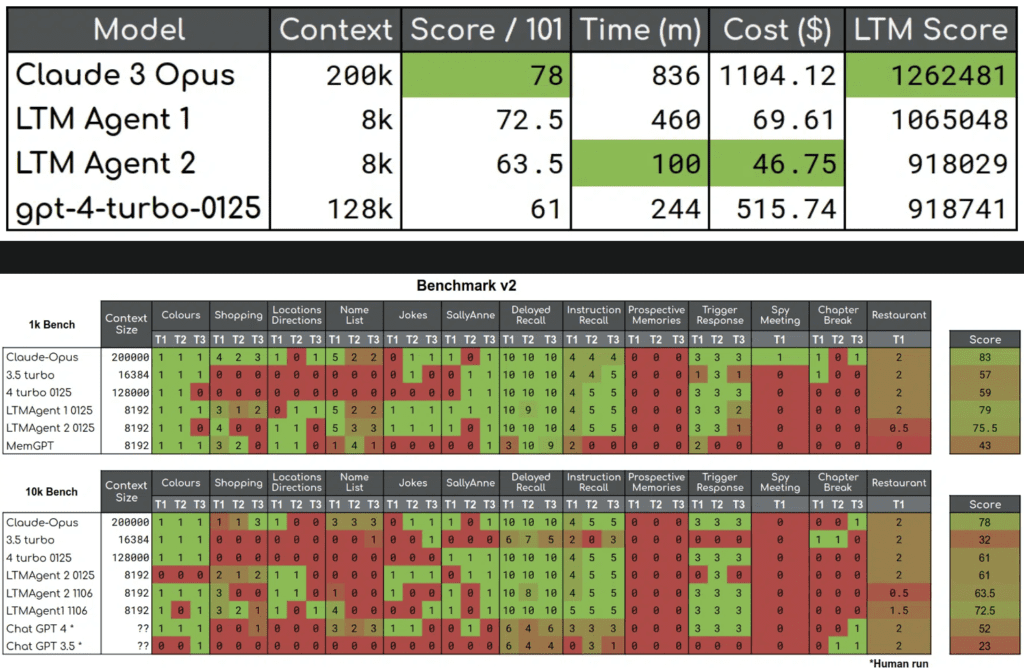
GoodAI’s commitment to developing AI agents capable of continual and lifelong learning takes a significant leap forward with the latest updates to the LTM Benchmark. This suite of tests is designed to assess the Long-Term Memory (LTM) capabilities of any conversational AI agent, providing a comprehensive measure of an AI’s ability to handle extended interactions.
Enhancements in Benchmark Methodology
The LTM Benchmark at GoodAI serves as a crucial tool for gauging the progress and effectiveness of AI agents in handling complex, long-duration conversations that mimic real-life interactions. Unlike previous iterations, the latest benchmark incorporates dynamic tests that are not only more aligned with natural human conversation but also allow for adjustments based on the AI’s responses. This shift from static to dynamic testing represents a major improvement in simulating real-world applications, where AI agents must respond to evolving situations.
Technical Innovations in Testing
One of the standout features in the new benchmark is the introduction of percentage waits, which allows tests to halt until a specified portion of other tasks has been completed. This method provides a more nuanced approach to managing the flow of the conversation, reflecting scenarios that AI may face in practical applications, such as digital assistants or customer service bots. By implementing percentage waits, GoodAI enhances the naturalness and dynamism of the tests, moving away from the rigidity of predefined scripts.
Moreover, the benchmark now includes tasks that require a higher degree of information integration and memory retrieval. These tasks are designed to challenge the AI’s ability to not just recall information but to synthesize and apply it in context. For instance, the Spy Meeting task involves the AI deciphering cryptic messages over a sequence of interactions, testing its ability to maintain and utilize key information over extended periods.
Future Directions and Community Involvement
With each iteration, GoodAI aims to push the boundaries of what conversational AI can achieve, particularly in terms of memory and adaptability. The open-source nature of the LTM Benchmark encourages contributions from the wider AI research community, fostering a collaborative environment for innovation. Future enhancements will likely focus on refining the integration of tasks and expanding the scope of tests to cover more complex and varied scenarios.
GoodAI’s latest release of the LTM Benchmark not only signifies advancements in AI testing but also sets a higher standard for the development of AI agents that can effectively participate in our daily lives. As AI continues to integrate into various sectors, the improvements in LTM capabilities will play a crucial role in ensuring these technologies can perform reliably and contextually in complex, long-term interactions.