Unveiling New Possibilities in Text-Image Comprehension and Composition
- Enhanced Vision-Language Comprehension: IXC-2.5 supports ultra-high resolution and fine-grained video understanding, along with multi-turn multi-image dialogue.
- Extended Contextual Input and Output: Utilizing RoPE extrapolation, IXC-2.5 can handle up to 96K long contexts, enhancing its ability to manage extensive input and output data.
- Versatile Applications: The model excels in crafting webpages and composing high-quality text-image articles, outperforming many open-source state-of-the-art models.
InternLM-XComposer-2.5 (IXC-2.5) represents a significant leap in the realm of large vision-language models (LVLMs). Developed with the capability to handle long-contextual input and output, IXC-2.5 is poised to transform text-image comprehension and composition tasks. Its robust performance is particularly noteworthy, achieving capabilities comparable to GPT-4V with a considerably smaller 7B large language model (LLM) backend.
Enhanced Vision-Language Comprehension
One of the most striking advancements in IXC-2.5 is its ability to understand ultra-high resolution images and fine-grained videos. This enables the model to analyze visual content with unprecedented detail, making it highly effective in applications that demand precise visual comprehension. Additionally, IXC-2.5 supports multi-turn, multi-image dialogue, allowing it to engage in complex conversations involving multiple images over extended interactions.
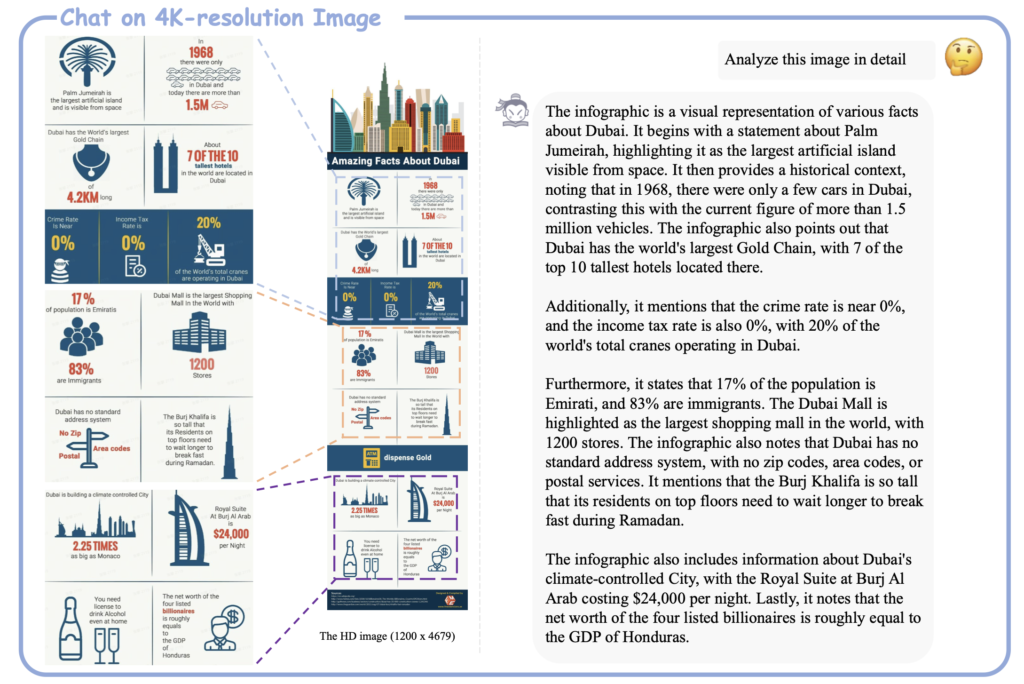
Extended Contextual Input and Output
The model’s capacity to handle long contexts is a game-changer. Trained with 24K interleaved image-text contexts and extendable to 96K contexts via RoPE extrapolation, IXC-2.5 excels in tasks that require extensive input and output contexts. This makes it particularly suitable for applications involving large datasets or detailed analysis, where maintaining context over a long sequence is crucial.
Versatile Applications
IXC-2.5 is not just a comprehension model; it also excels in composition. Leveraging additional LoRA parameters, it can craft webpages and compose high-quality text-image articles. This dual capability enhances its utility, making it a powerful tool for both understanding and generating complex multimedia content.
In comprehensive evaluations across 28 benchmarks, IXC-2.5 has outperformed existing open-source state-of-the-art models on 16 benchmarks, and competes closely with leading models like GPT-4V and Gemini Pro on 16 key tasks. This performance underscores the model’s efficiency and versatility, achieved with a relatively modest LLM backend.
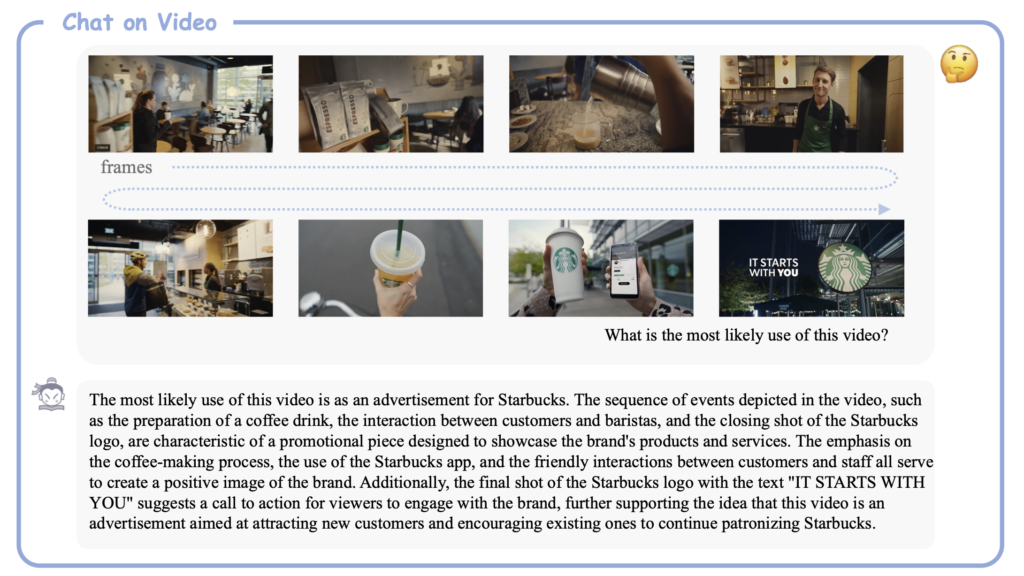
Future Directions and Potential Applications
InternLM-XComposer-2.5 sets a promising research direction, particularly in contextual multi-modal environments. Future developments could include long-context video understanding, such as analyzing full-length movies or maintaining long-term interaction histories. These advancements would significantly enhance real-world applications, providing better assistance in various fields, from content creation to advanced data analysis.
Acknowledgments
The development of IXC-2.5 has been supported by contributions from the academic community, notably Prof. Chao Zhang from Tsinghua University, whose suggestions regarding audio models and tools have been invaluable.
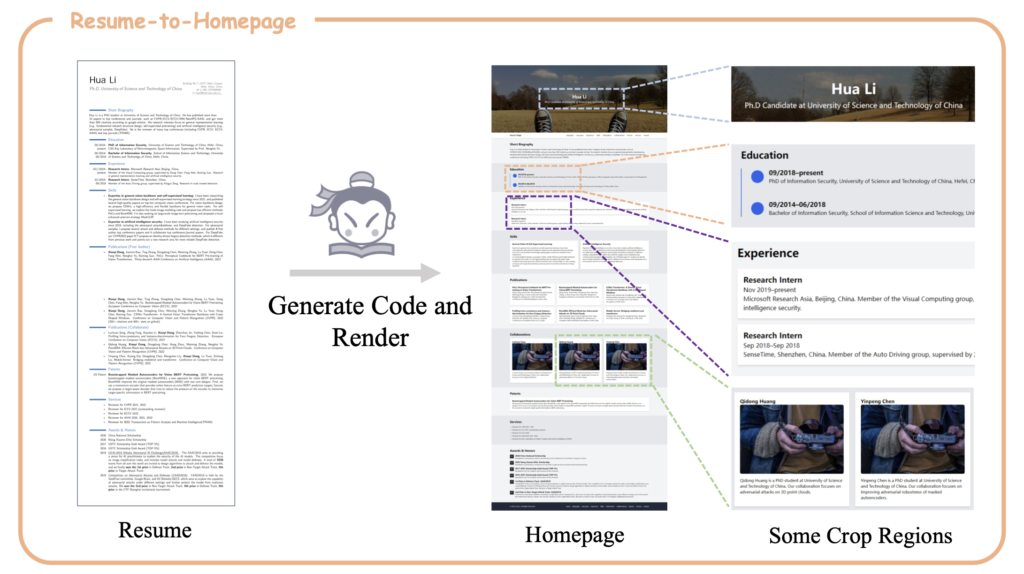
InternLM-XComposer-2.5 stands at the forefront of vision-language models, offering a versatile and powerful tool for comprehending and creating complex multimedia content. Its ability to handle extended contexts and deliver high-quality results marks a significant milestone in the advancement of AI-driven text-image models.