A New Benchmark Tests AI’s Ability to Answer Real-Time Visual Questions
- Introducing LIVEVQA – A groundbreaking dataset of 3,602 visual questions sourced from live news, designed to test AI’s ability to understand and reason about real-time visual information.
- AI’s Biggest Challenge Yet – Even top models like GPT-4o and Gemini struggle with multi-hop visual reasoning, revealing critical gaps in live knowledge retrieval.
- The Future of AI Understanding – While search tools help, true real-time visual comprehension remains an unsolved problem, pointing to exciting research opportunities ahead.
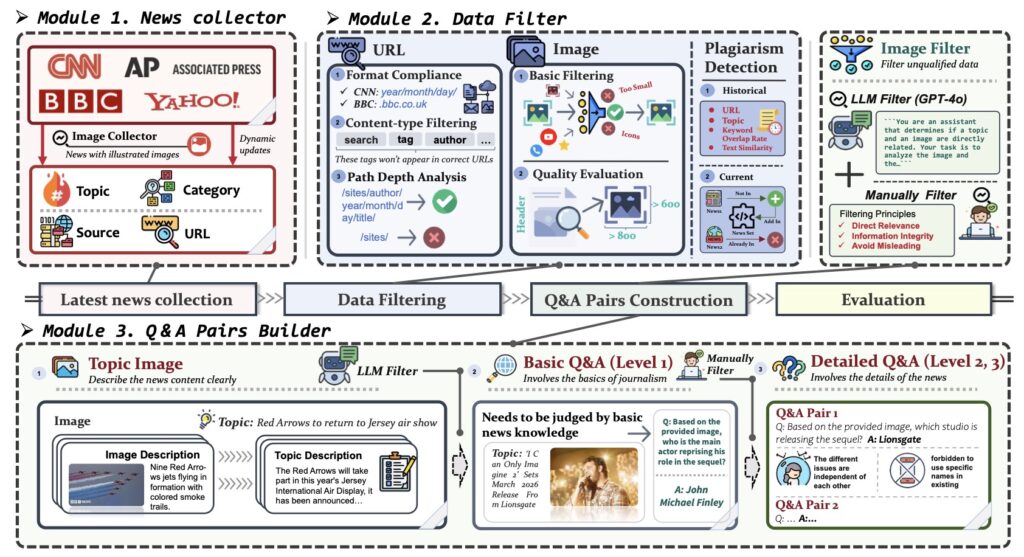
In today’s fast-moving digital world, news and events unfold in real-time across the globe. AI systems must keep up—not just with text but with the visual content that shapes our understanding of current events. While large language models (LLMs) have made impressive strides in processing live textual data (especially when paired with search engines), a crucial question remains: Can AI systems truly understand live visual knowledge?
Enter LIVEVQA, a new benchmark designed to push AI’s limits in real-time visual comprehension. Unlike traditional datasets that rely on static images, LIVEVQA pulls the latest news from six major global platforms, ensuring that models can’t rely on memorized data—they must truly see, interpret, and reason about fresh visual content.
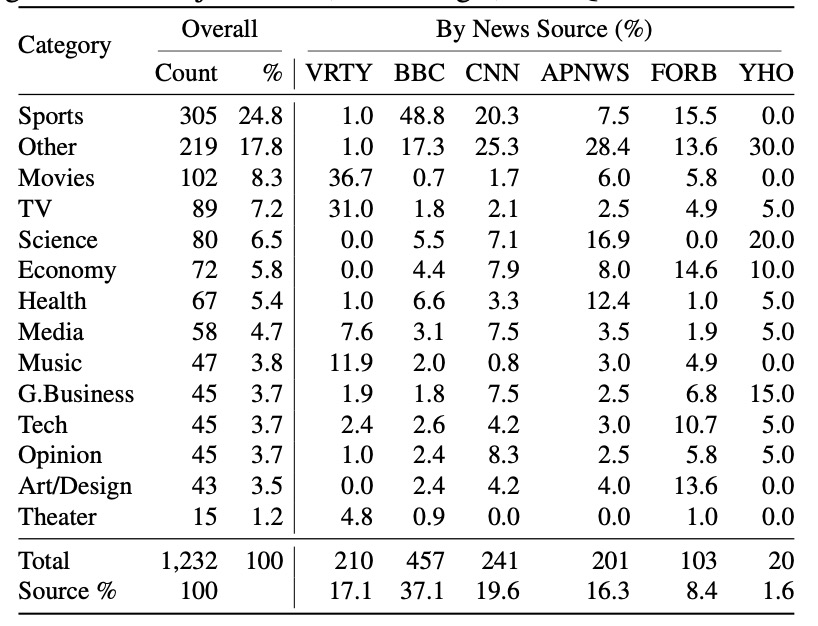
What Makes LIVEVQA Unique?
LIVEVQA isn’t just another visual question-answering dataset. It’s built with three core principles:
- Strict Temporal Filtering – Only the most recent news is included, forcing AI to retrieve rather than recall.
- Automated Ground Truth with Human Oversight – High-quality annotations ensure meaningful, challenging questions.
- Authentic Image-Text Pairs – Every question is tied to real-world news, demanding deep visual-textual reasoning.
The dataset spans 14 news categories, from politics to sports, and includes 1,233 news articles with 3,602 questions—ranging from basic visual recognition to complex multi-hop reasoning.
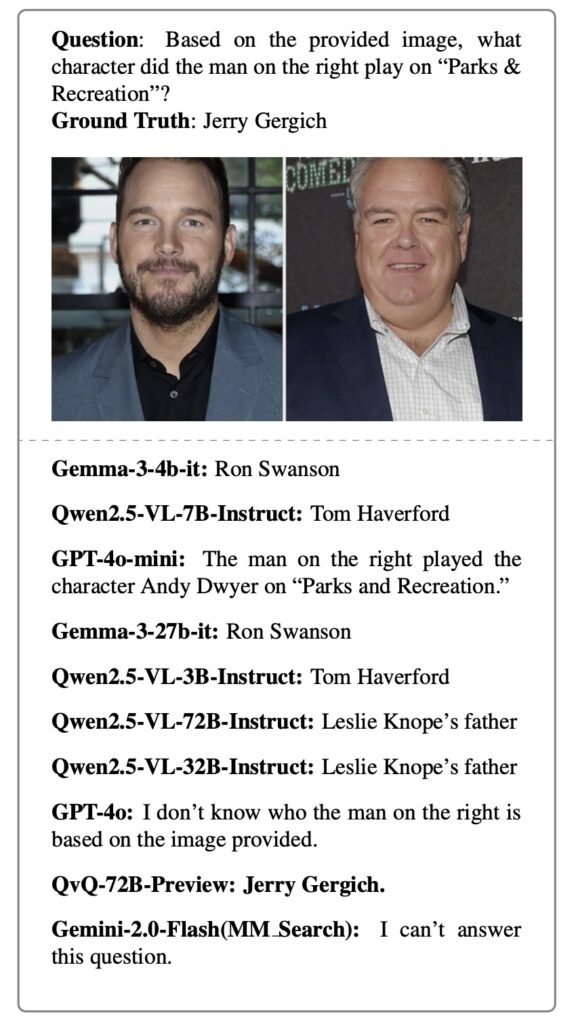
How Do Today’s AI Models Perform?
The results are revealing. Researchers evaluated 15 state-of-the-art multimodal models, including GPT-4o, Gemma-3, and the Qwen-2.5-VL family. While stronger models generally performed better, multi-hop visual questions proved a major stumbling block.
Key findings:
- Search Tools Help, But Aren’t Enough – Models with internet access (like those using Google Search or GUI-based image search) saw a performance boost, but still fell short on complex visual reasoning.
- Text vs. Vision Gap – Even models excelling at live text-based questions struggled when visual knowledge was required.
- Bigger Isn’t Always Better – While larger models (e.g., 72B parameters) had an edge, architecture and reasoning capabilities mattered more than sheer size.
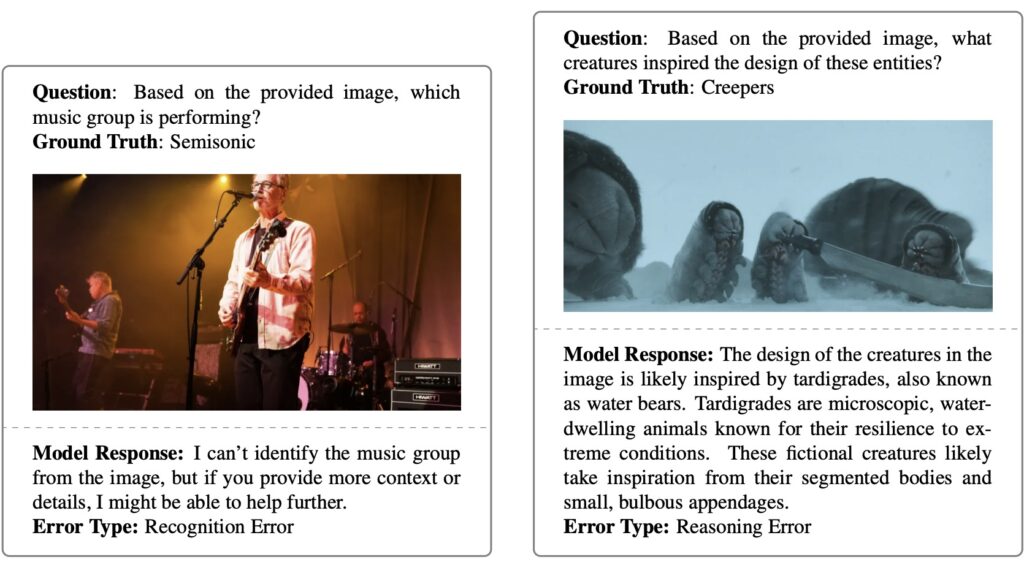
Why Does This Matter?
The implications are vast. From real-time fact-checking to automated news summarization, AI’s ability to process live visuals is crucial. Yet, LIVEVQA shows that today’s systems still lack robust visual reasoning—especially when connecting multiple pieces of information (e.g., identifying a person in an image and linking them to a recent event).
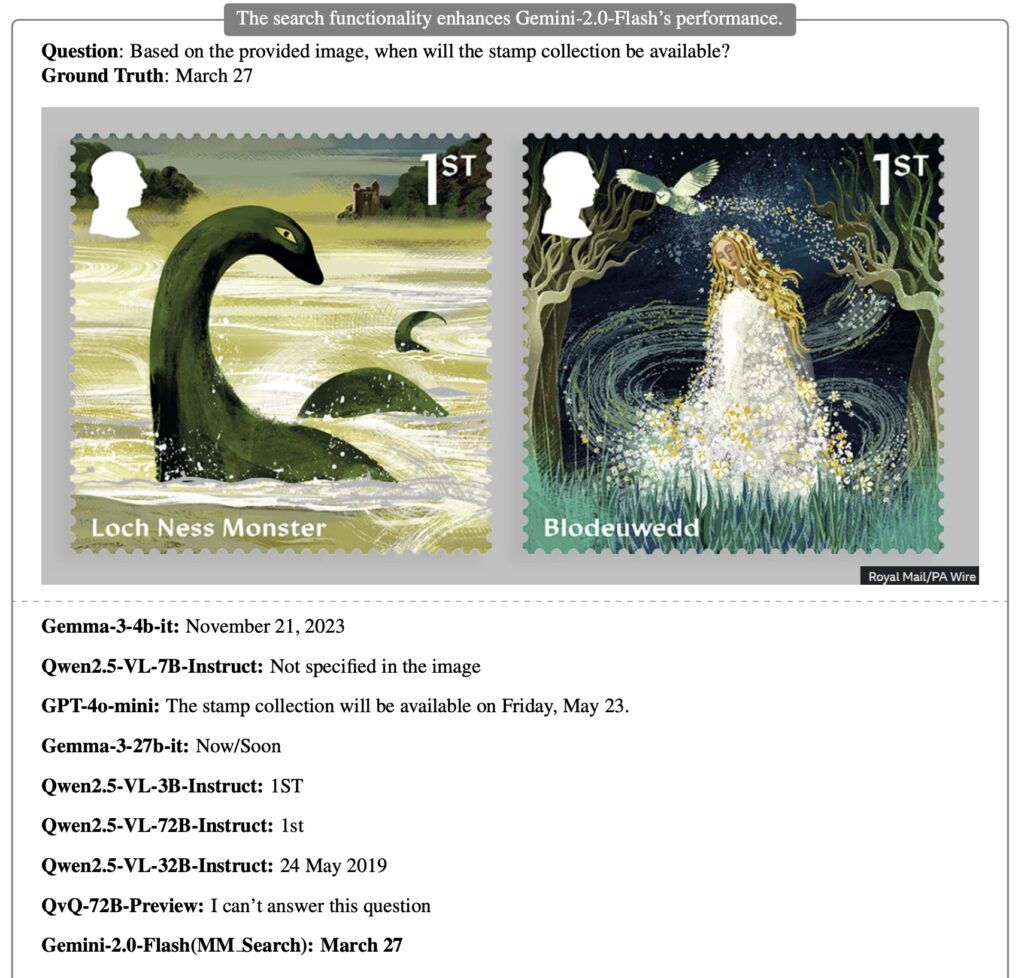
Where AI Needs to Improve
The study highlights three key areas for future research:
- Better Visual Retrieval – AI needs more advanced ways to fetch and verify live images.
- Stronger Multi-Hop Reasoning – Models must improve at connecting visual and textual clues dynamically.
- Real-Time Learning – Systems should adapt to new visual knowledge without extensive retraining.
As AI continues evolving, benchmarks like LIVEVQA will be essential in measuring progress—and ensuring that AI doesn’t just read the news but truly sees and understands it.
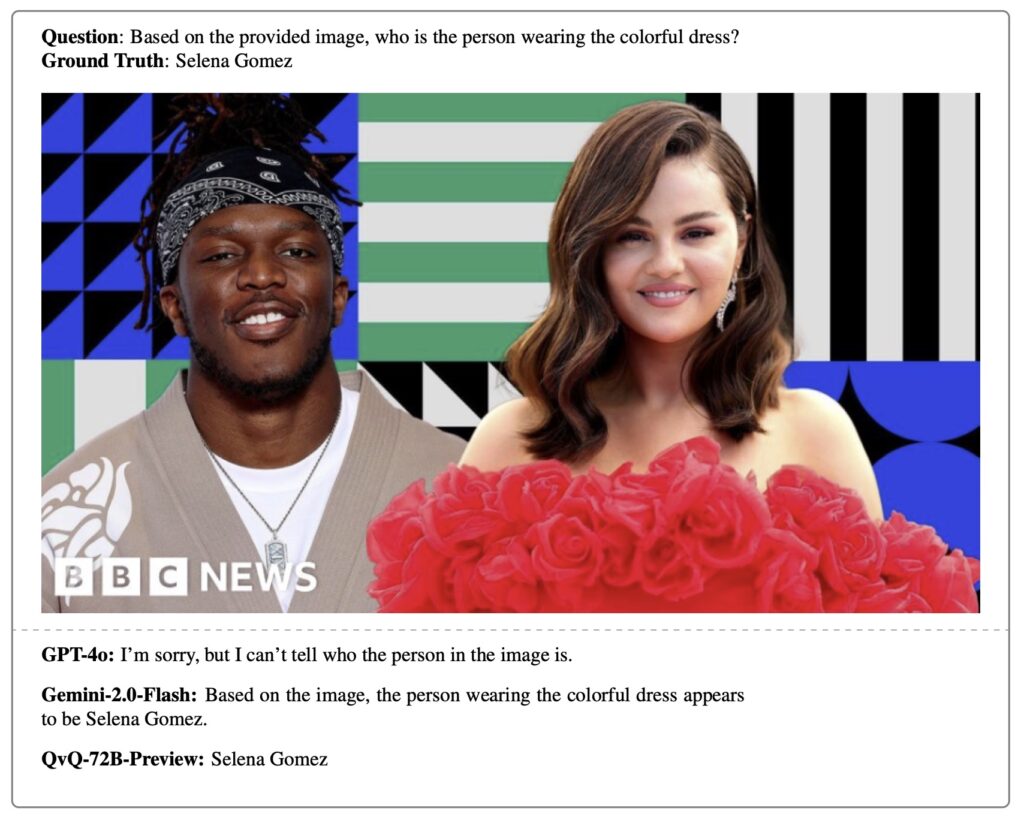