How MeshFormer pushes the boundaries of 3D reconstruction with smart architecture, fewer resources, and better performance.
- Efficient 3D Mesh Generation: MeshFormer introduces a unified, single-stage model that uses explicit 3D structures and minimal resources—just 8 GPUs—to generate high-quality 3D meshes.
- Leveraging 2D Diffusion Models: By combining 2D models to predict normal maps and multi-view images, MeshFormer refines geometry, enabling faster and more accurate 3D reconstructions from sparse input views.
- Real-World Applications: MeshFormer sets a new standard for creating textured meshes with fine details, ideal for rendering, simulations, and 3D printing—overcoming the challenges of prior models requiring extensive resources.
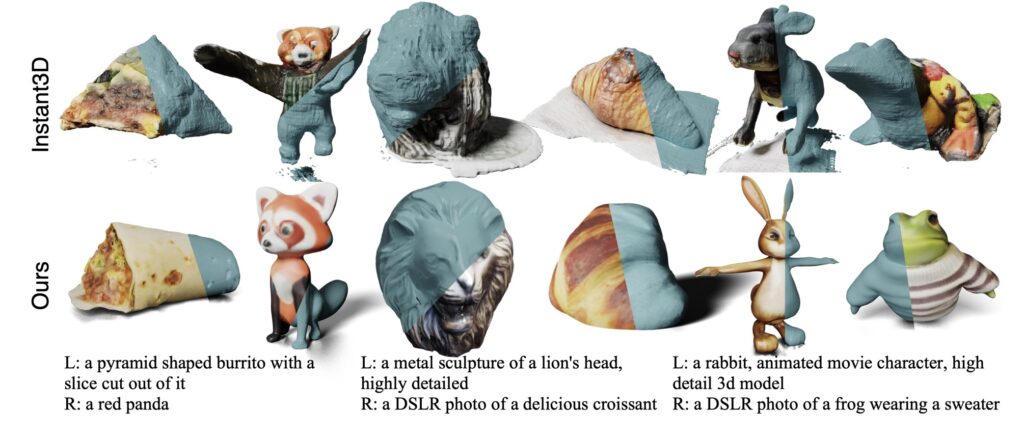
In the evolving world of 3D reconstruction, generating high-quality 3D meshes has traditionally required vast amounts of data, extensive GPU resources, and complex multi-stage processes. However, MeshFormer is setting a new precedent, offering an innovative model that efficiently produces detailed, textured 3D meshes using significantly fewer resources—without compromising on quality.
Efficiency Meets Innovation in 3D Reconstruction
At the heart of MeshFormer is a sparse-view reconstruction model that leverages explicit 3D structure and smart training supervision to deliver superior results. Instead of relying on large-scale triplane representation models that require hundreds of GPUs and expensive training processes, MeshFormer achieves its remarkable performance using only 8 GPUs, thanks to its advanced Efficient Frame Pruning (EFP) mechanism. This approach drastically reduces computational demand while still delivering high-quality meshes with fine-grained geometric details.
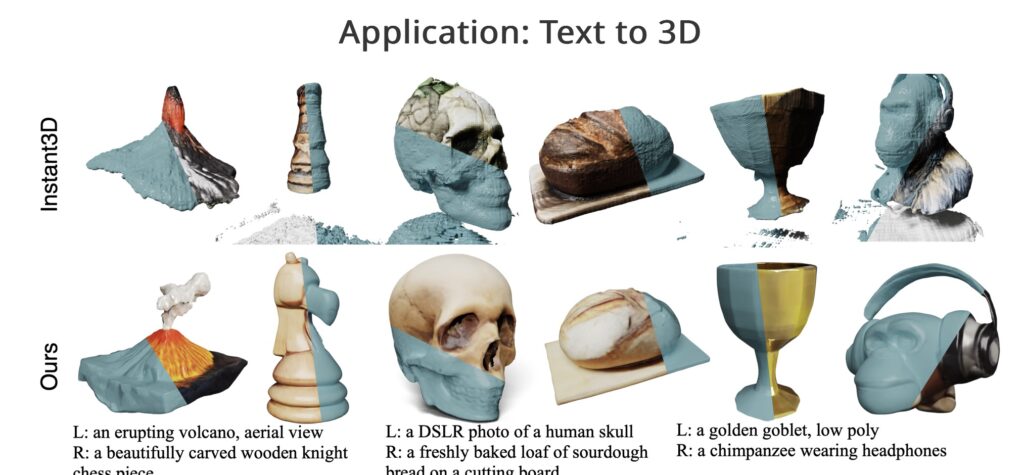
2D Diffusion Models: The Key to Realism
To further refine the process, MeshFormer taps into 2D diffusion models to predict normal maps and multi-view RGB images, which guide the geometry’s learning and help in generating precise, realistic 3D reconstructions. By utilizing Signed Distance Function (SDF) supervision, MeshFormer eliminates the need for complex multi-stage workflows, which have been a stumbling block for earlier methods. This integration allows for faster, more accurate single-image-to-3D and text-to-3D tasks, making the process highly accessible and scalable for real-world applications.
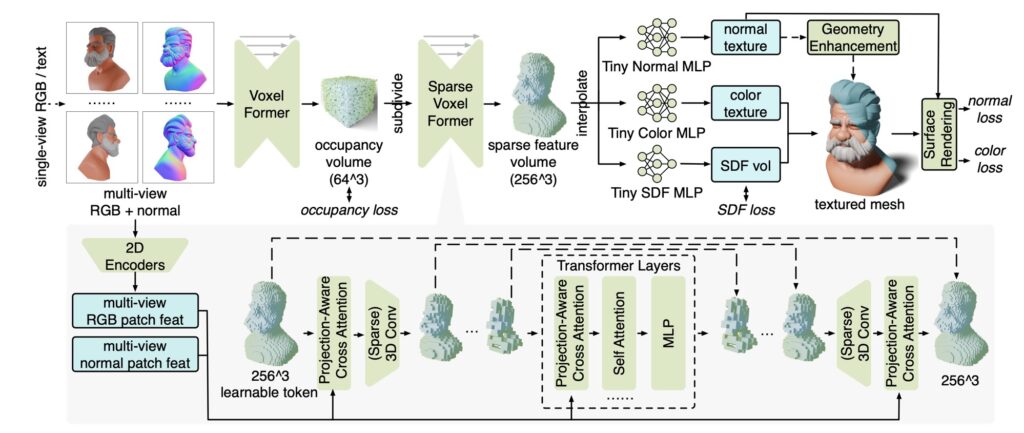
Real-World Impact: Fine Details and Textured Quality
MeshFormer isn’t just about speed and efficiency—it’s also about quality. Its ability to generate high-resolution, finely detailed 3D meshes makes it a game-changer for industries that rely on precise modeling, such as rendering, simulation, and 3D printing. MeshFormer consistently outperforms earlier models that struggled with texture and geometry quality, offering a practical and powerful solution for open-world object generation.
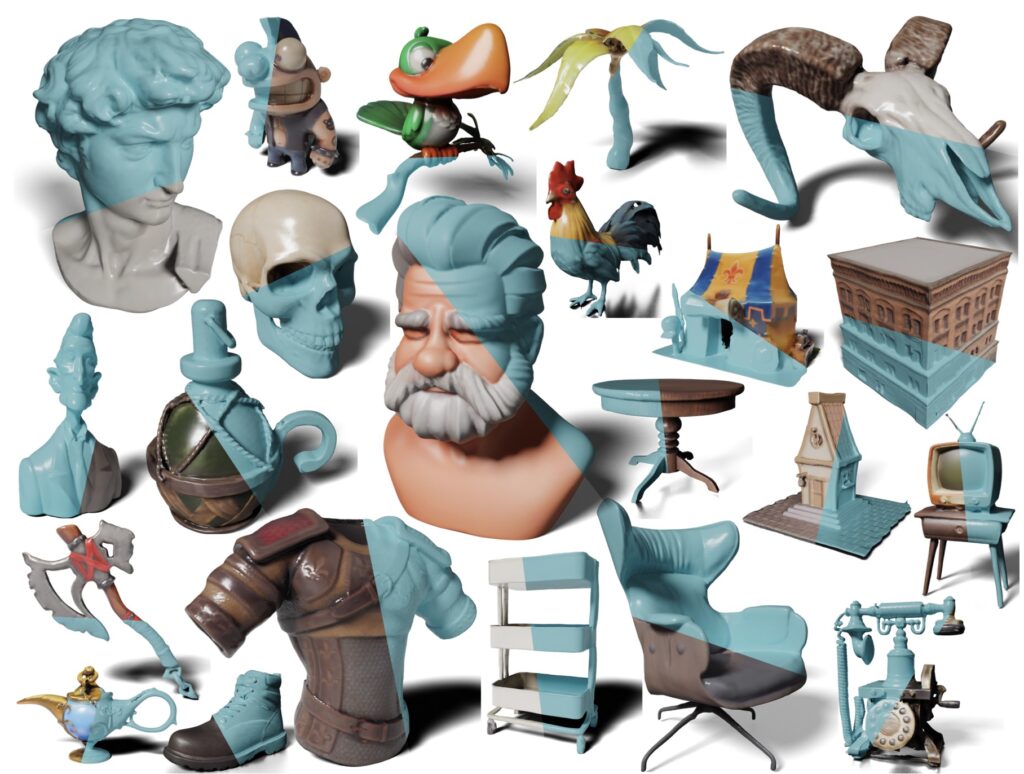
Looking Forward: Improving Robustness
While MeshFormer represents a major leap in 3D mesh generation, the model still faces challenges, particularly with generating consistent multi-view images—a crucial component for ensuring flawless reconstruction. Future developments aim to enhance MeshFormer’s robustness against imperfect predictions from 2D models, ensuring even greater reliability in diverse applications.
A New Era in 3D Reconstruction
MeshFormer has redefined what’s possible in the realm of 3D mesh generation, offering a smarter, faster, and more resource-efficient model that can achieve remarkable results with minimal computational power. With its combination of 2D diffusion models and innovative architecture, MeshFormer is poised to become the go-to solution for high-quality, real-time 3D reconstruction in numerous industries, paving the way for more accessible and scalable 3D asset creation.