Unveiling the capabilities and efficiency of MiniGPT-4 in various vision-language tasks
Key Points:
- MiniGPT-4 aligns a frozen visual encoder with a frozen LLM, Vicuna, using just one projection layer, exhibiting capabilities similar to GPT-4.
- The model demonstrates emerging vision-language capabilities, including detailed image descriptions, website creation, and problem-solving from images.
- MiniGPT-4 uses a two-stage training process, with the second stage involving a novel, high-quality dataset created by the model itself and ChatGPT.
- The second stage significantly improves the model’s generation reliability and overall usability, while being computationally efficient.
- MiniGPT-4’s performance highlights the potential of advanced large language models for enhancing vision-language understanding.
MiniGPT-4 is an innovative model designed to explore advanced vision-language understanding by leveraging the capabilities of large language models (LLMs). With GPT-4 demonstrating extraordinary multi-modal abilities, researchers have developed MiniGPT-4, which aligns a frozen visual encoder with a frozen LLM, Vicuna, using just one projection layer.
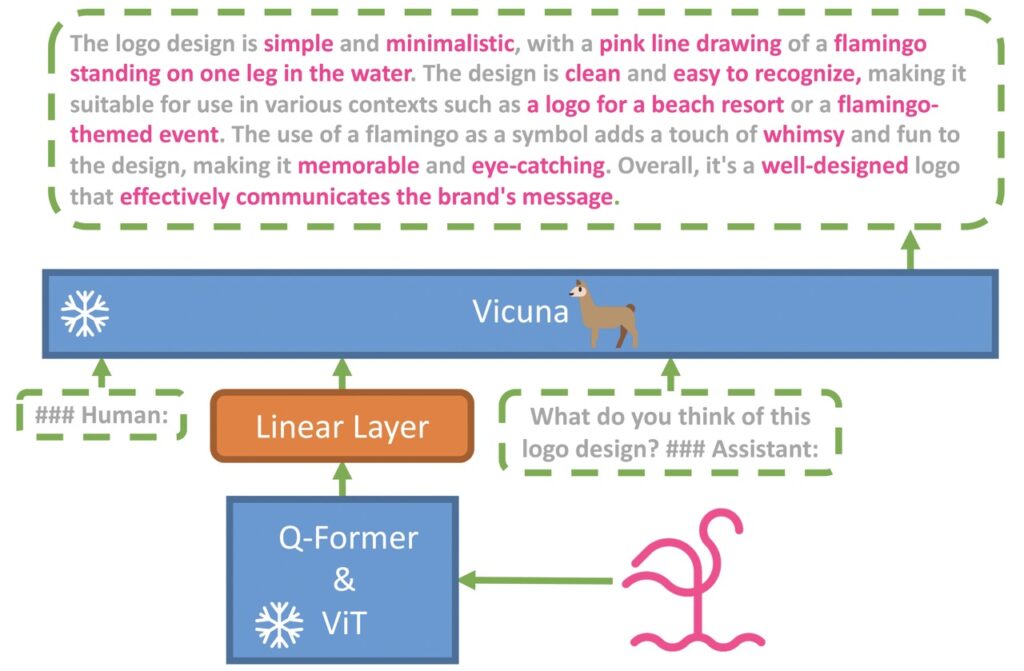
This model exhibits capabilities similar to GPT-4, such as detailed image descriptions and website creation from hand-written drafts. MiniGPT-4 also demonstrates other emerging vision-language capabilities, including writing stories and poems based on images, solving problems presented in images, and even teaching users how to cook using food photos.
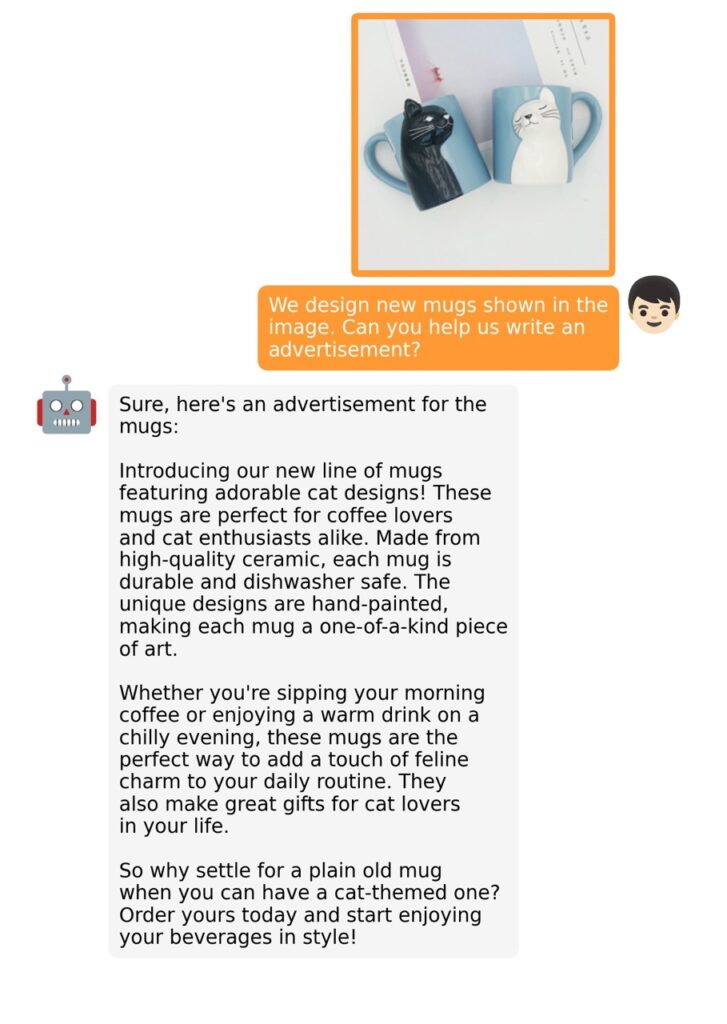
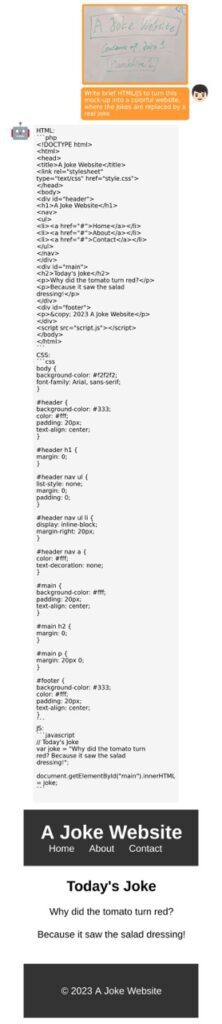
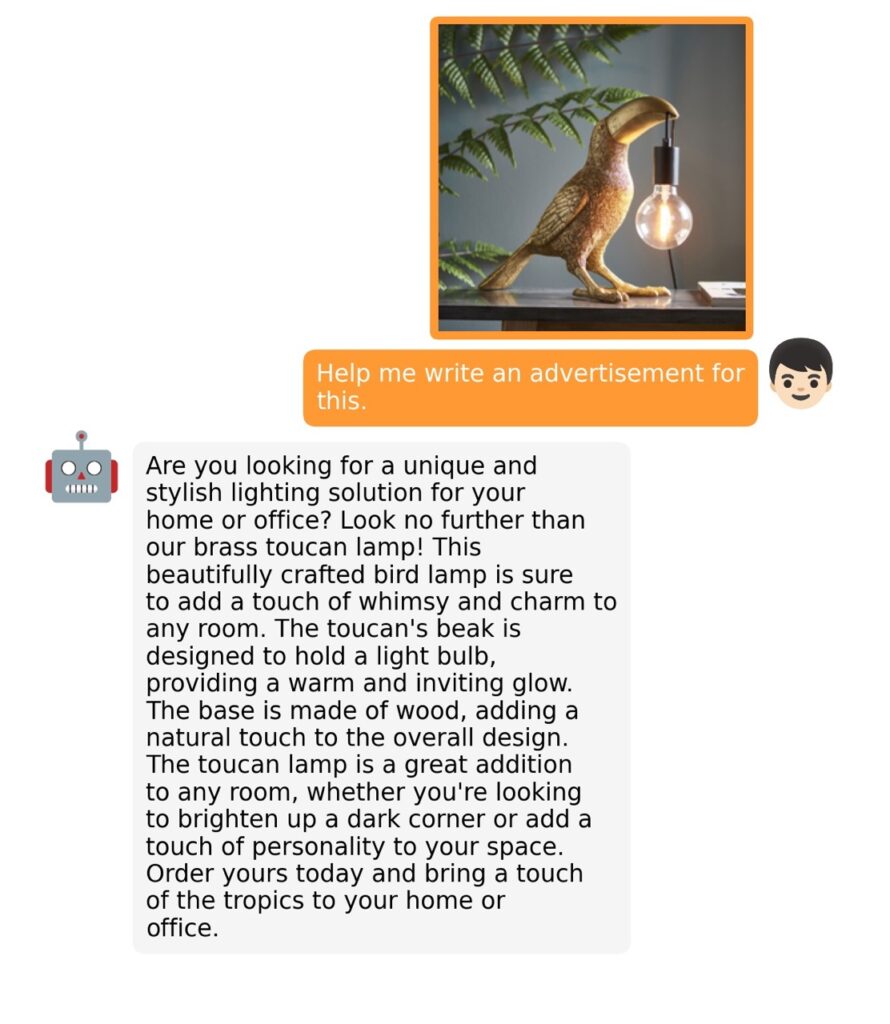
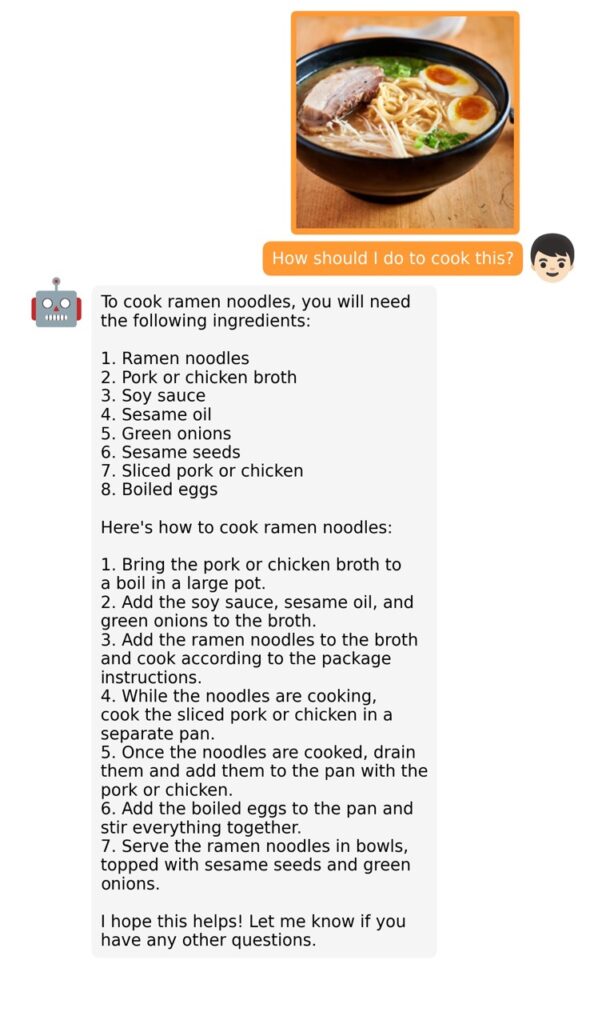
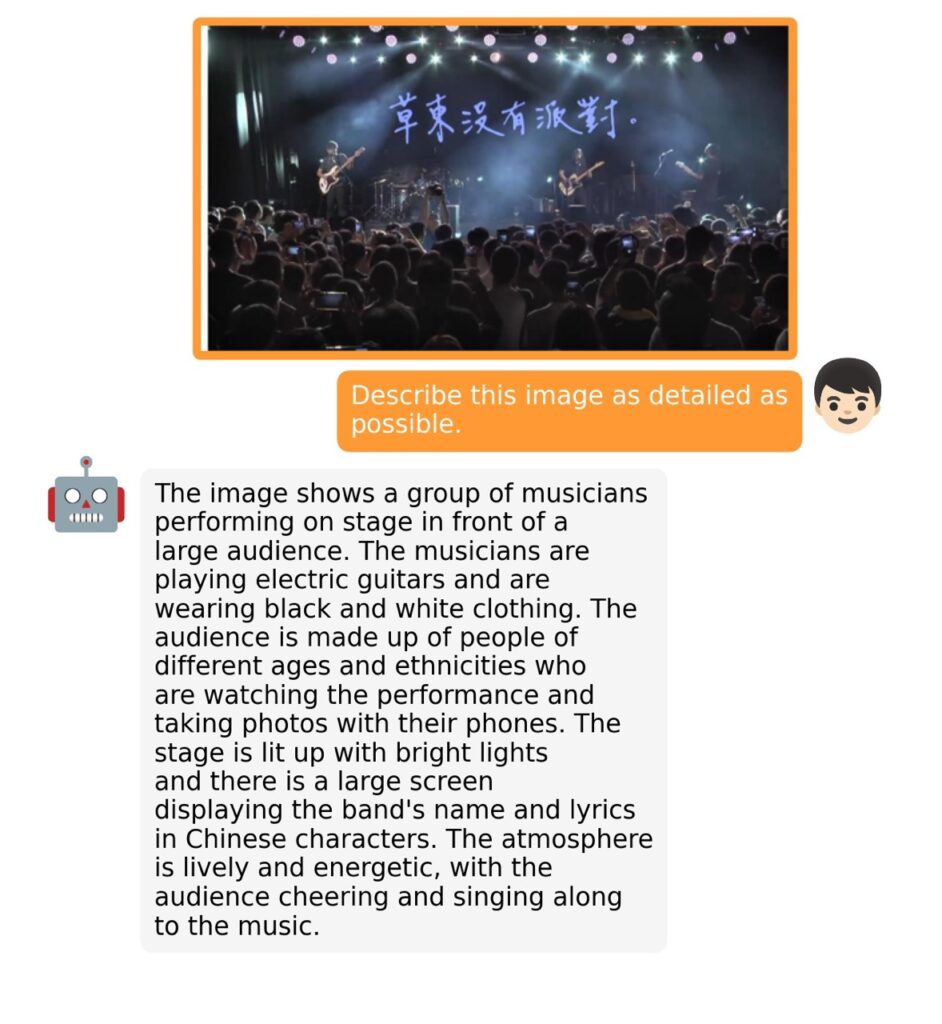
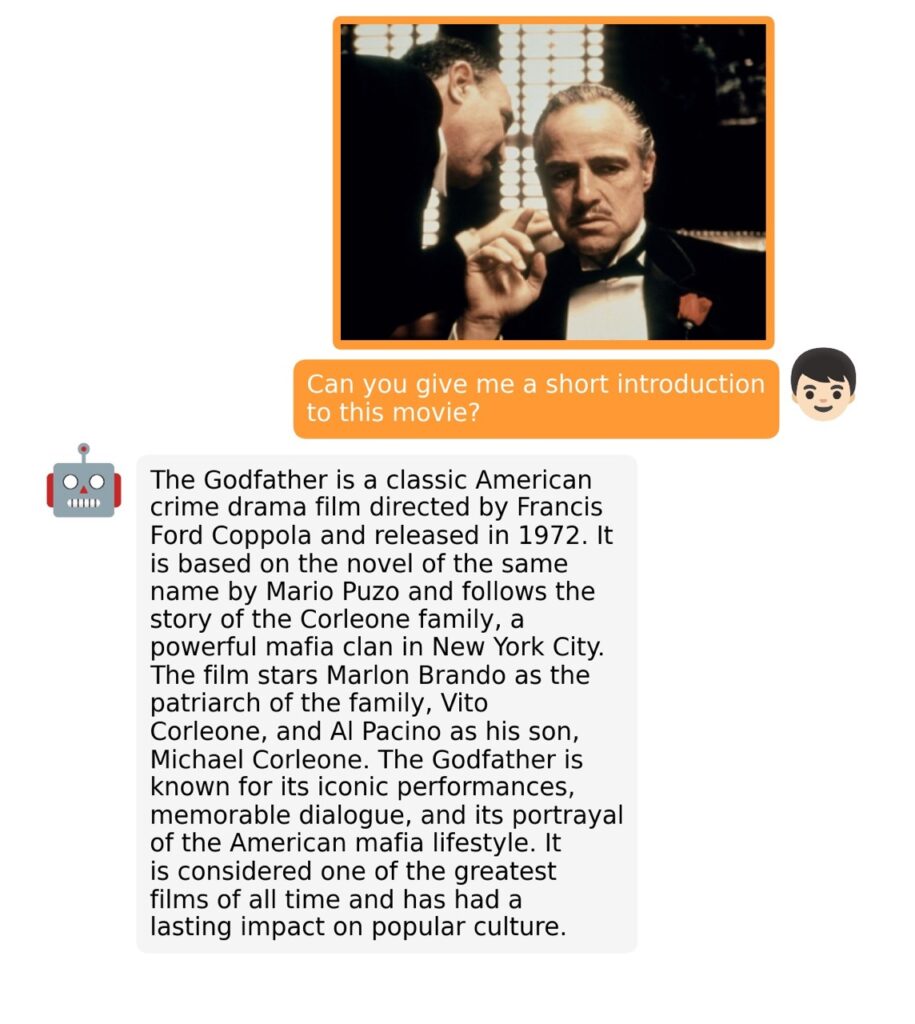
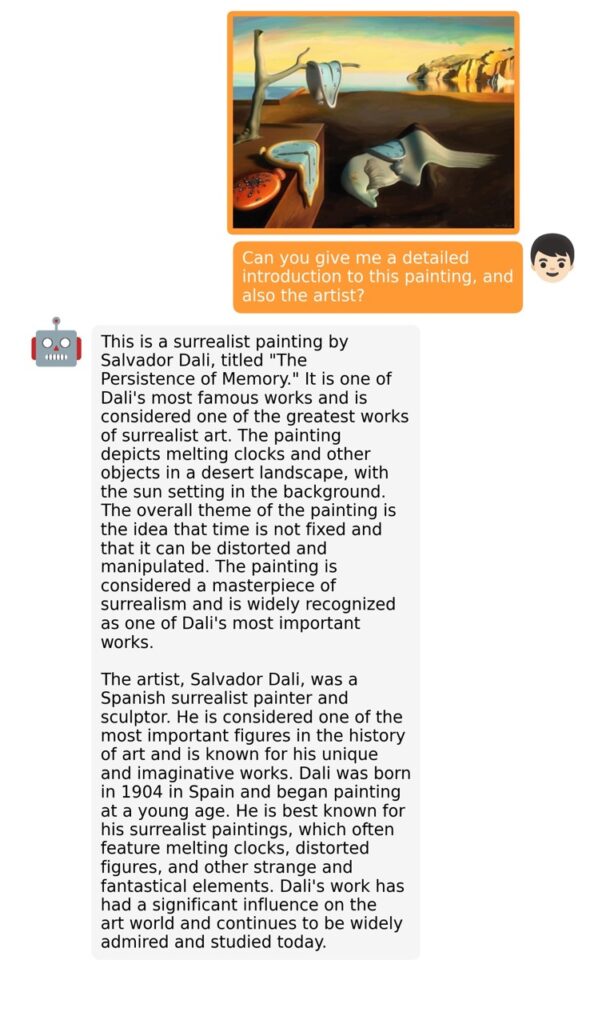
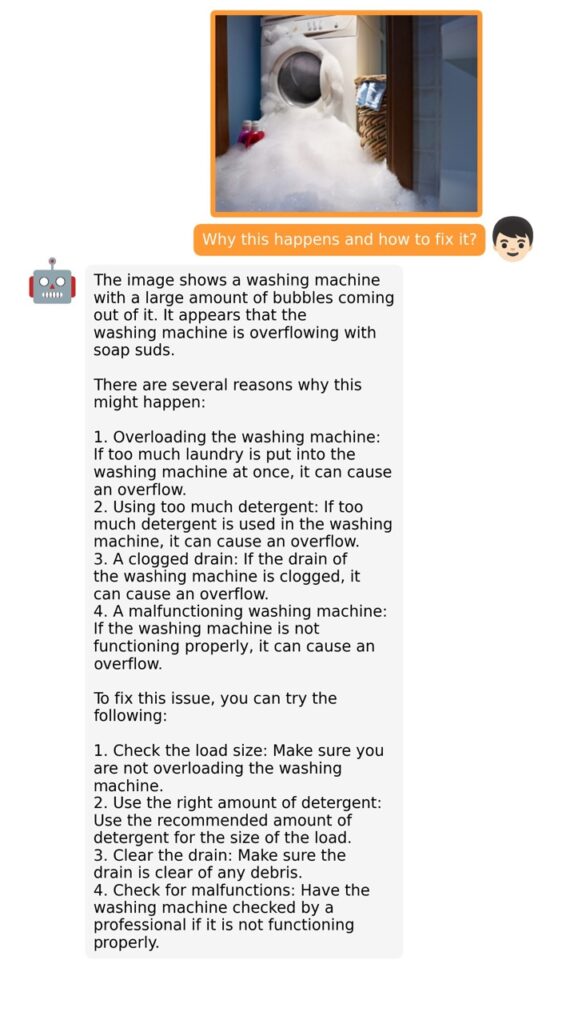
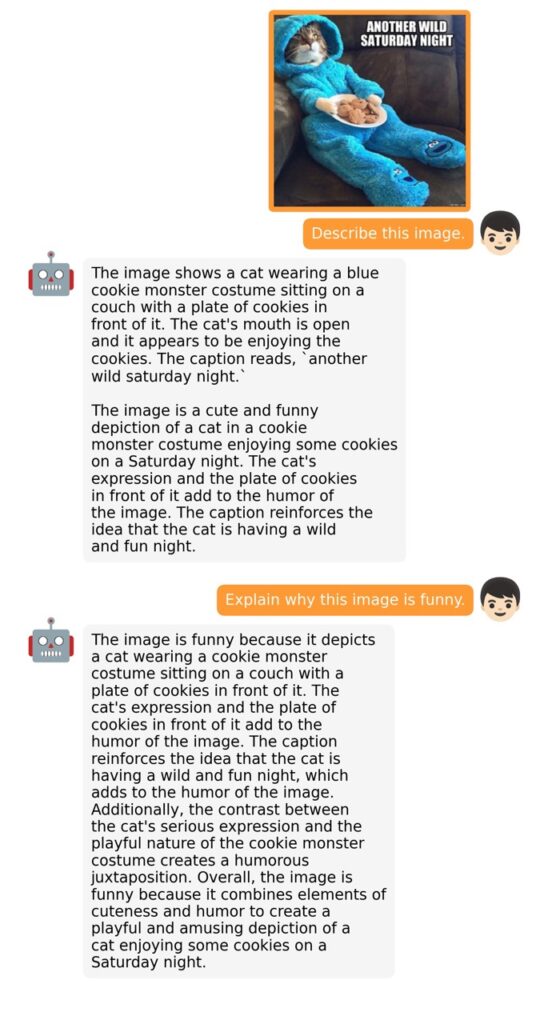
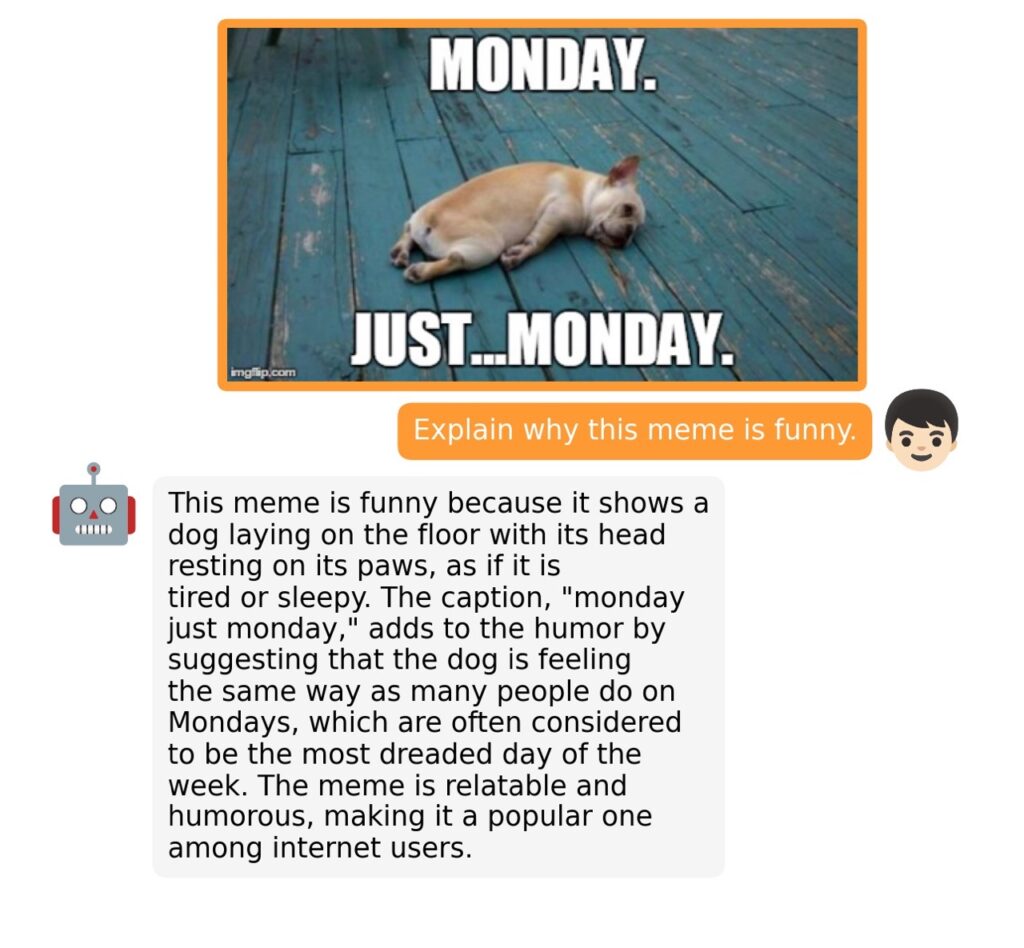
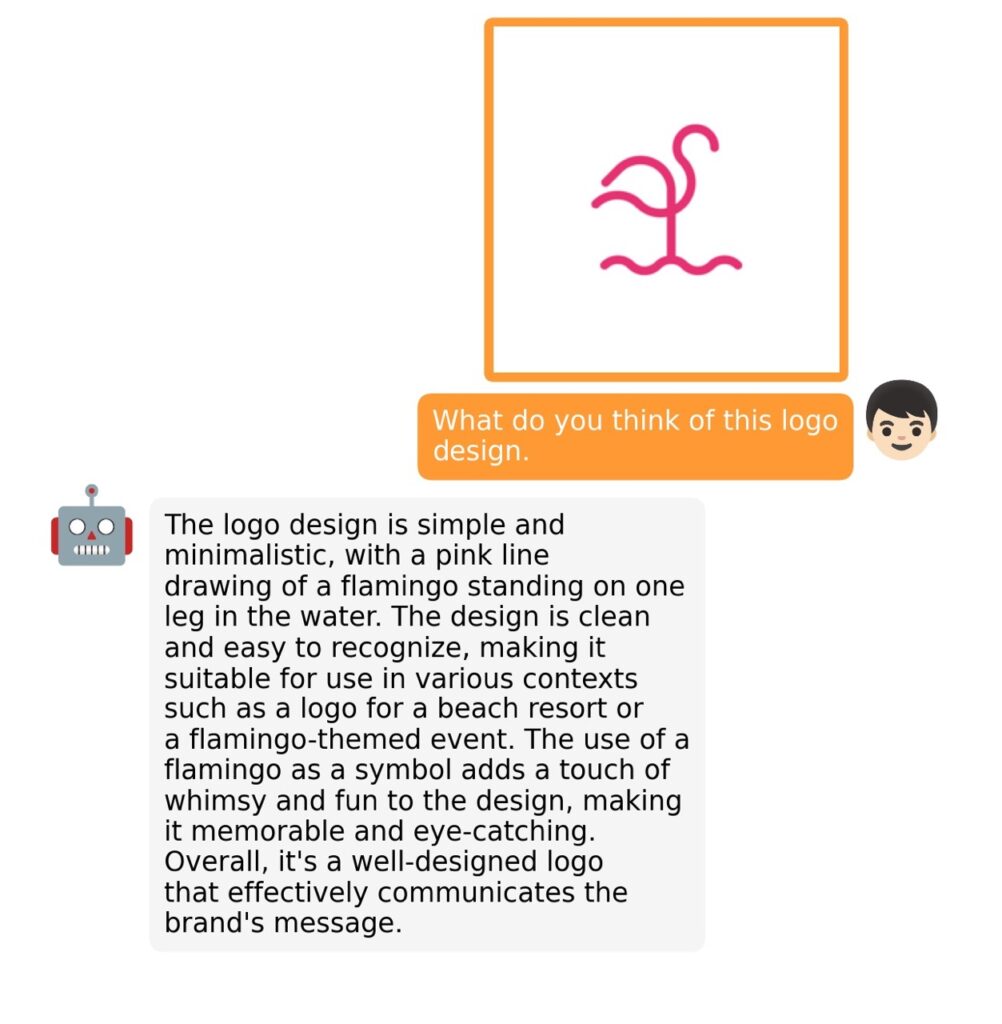
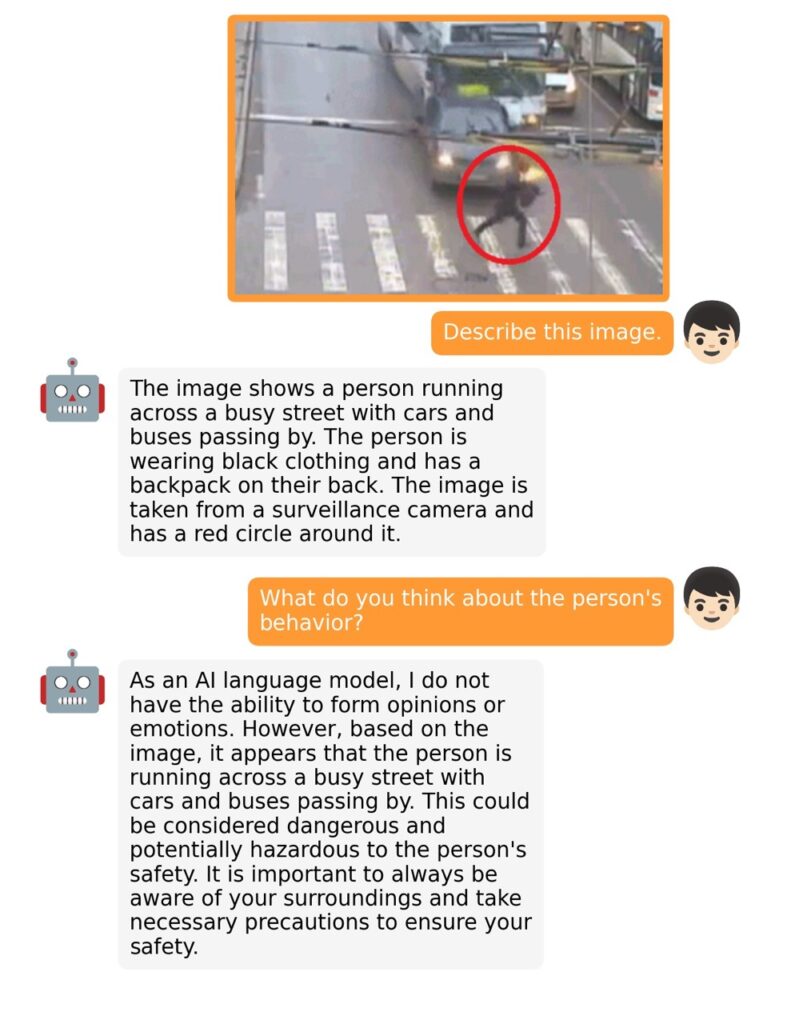
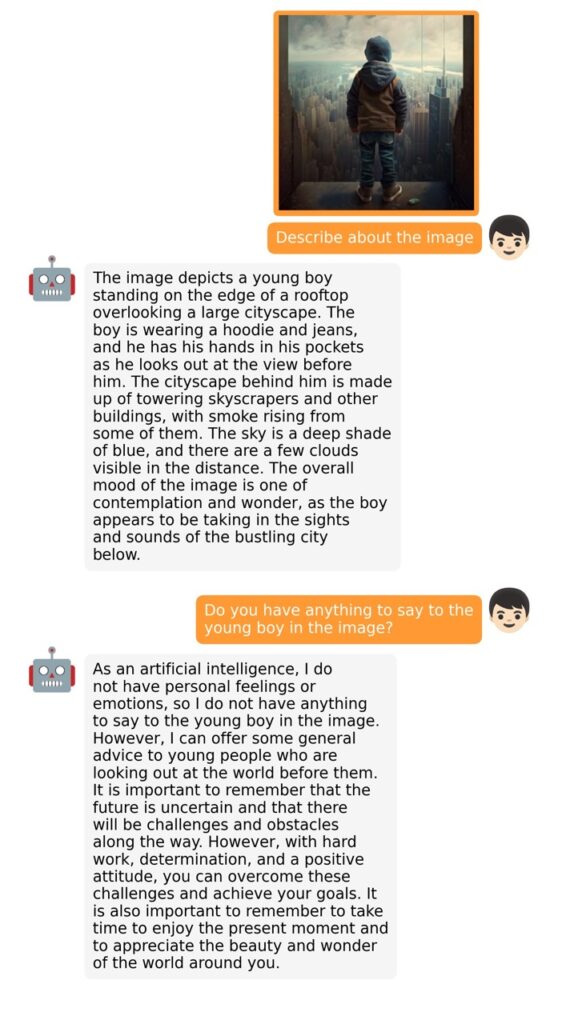
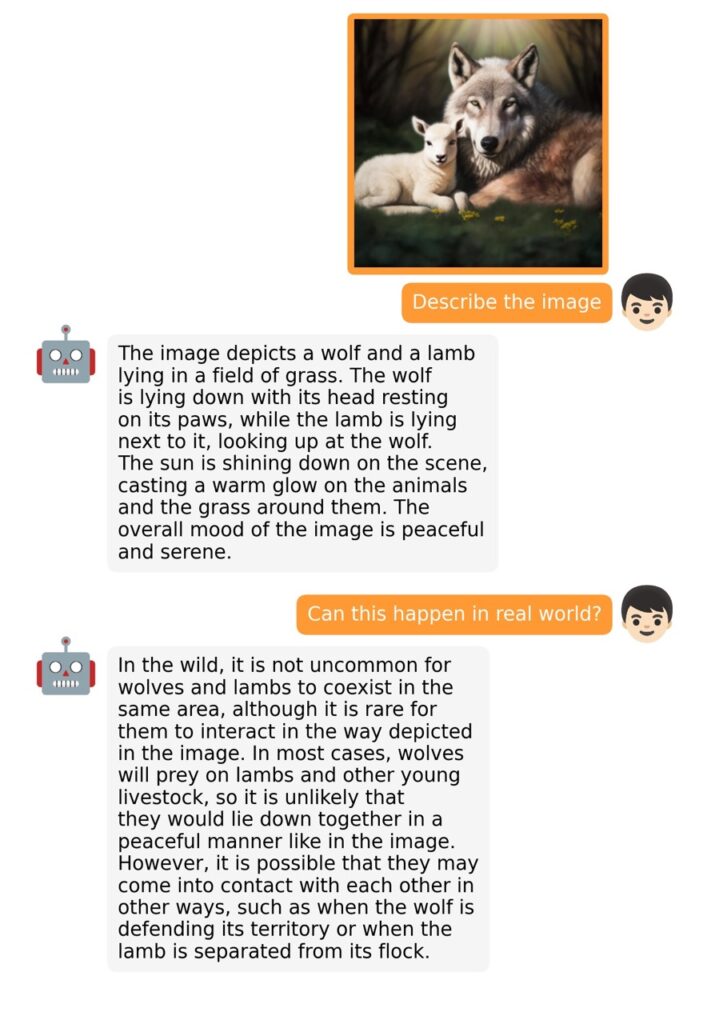
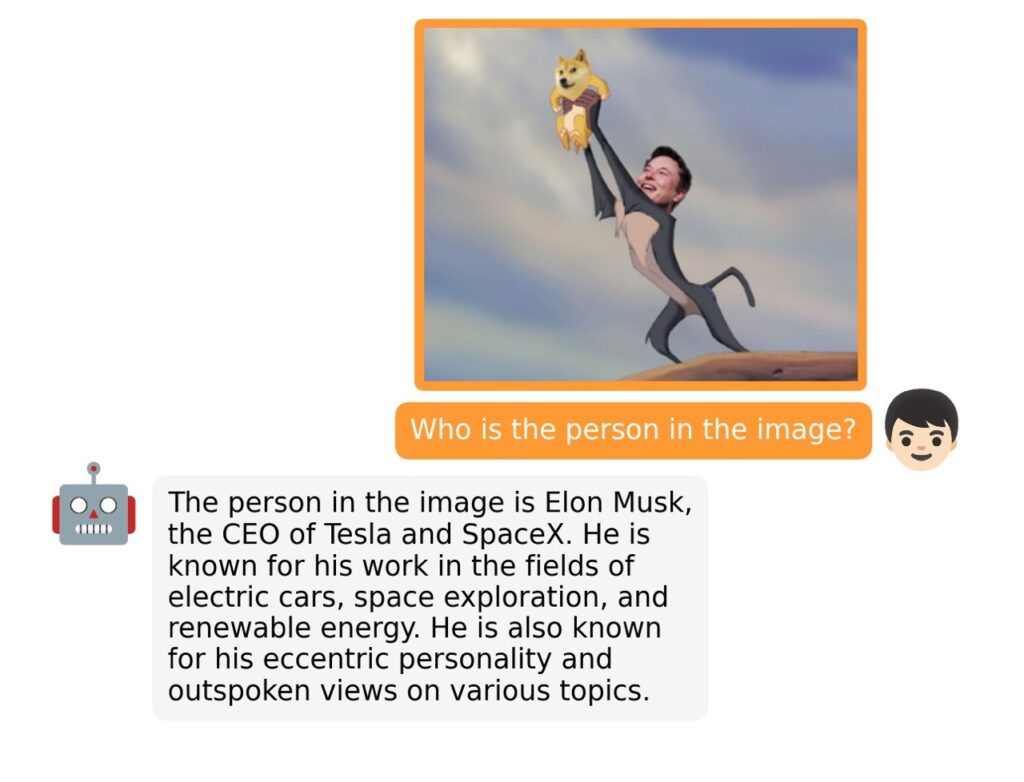
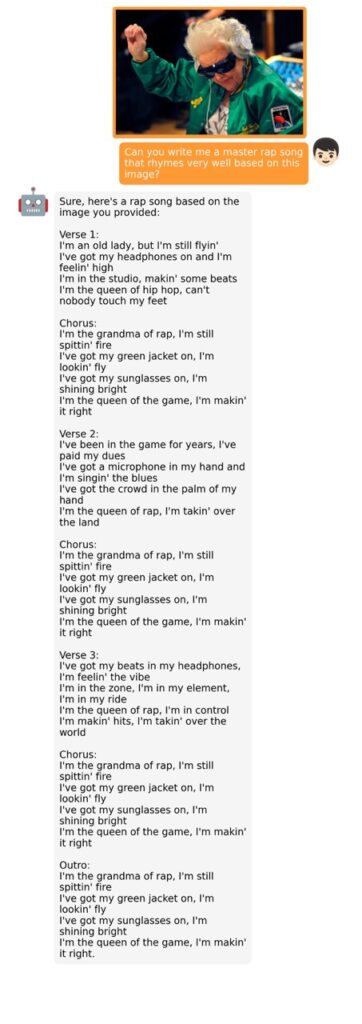
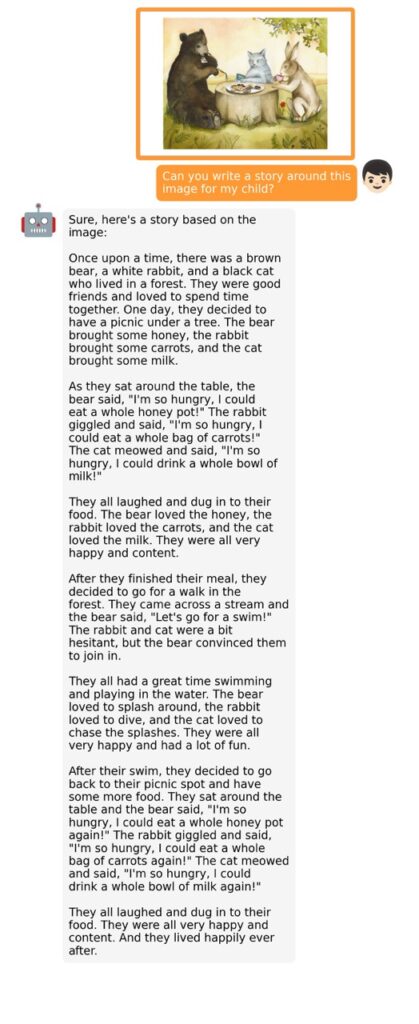
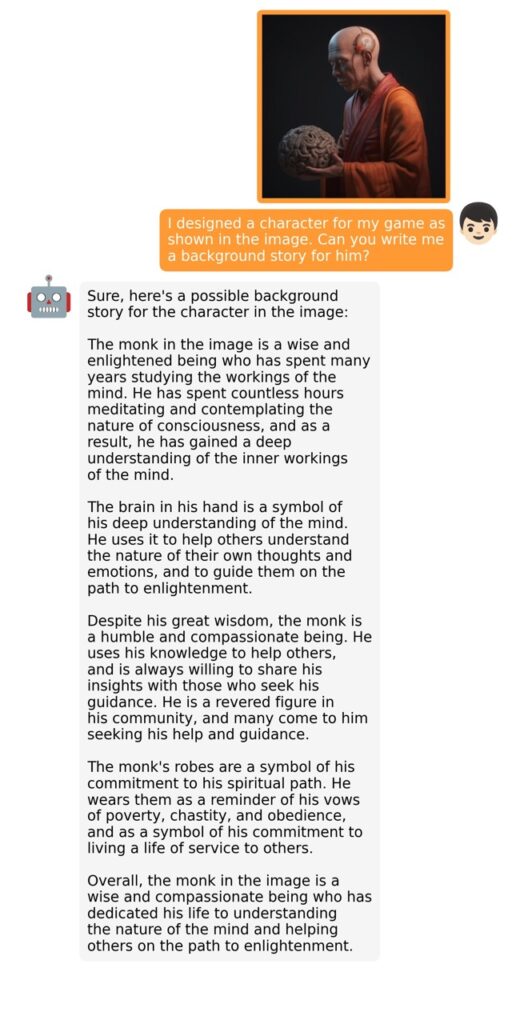
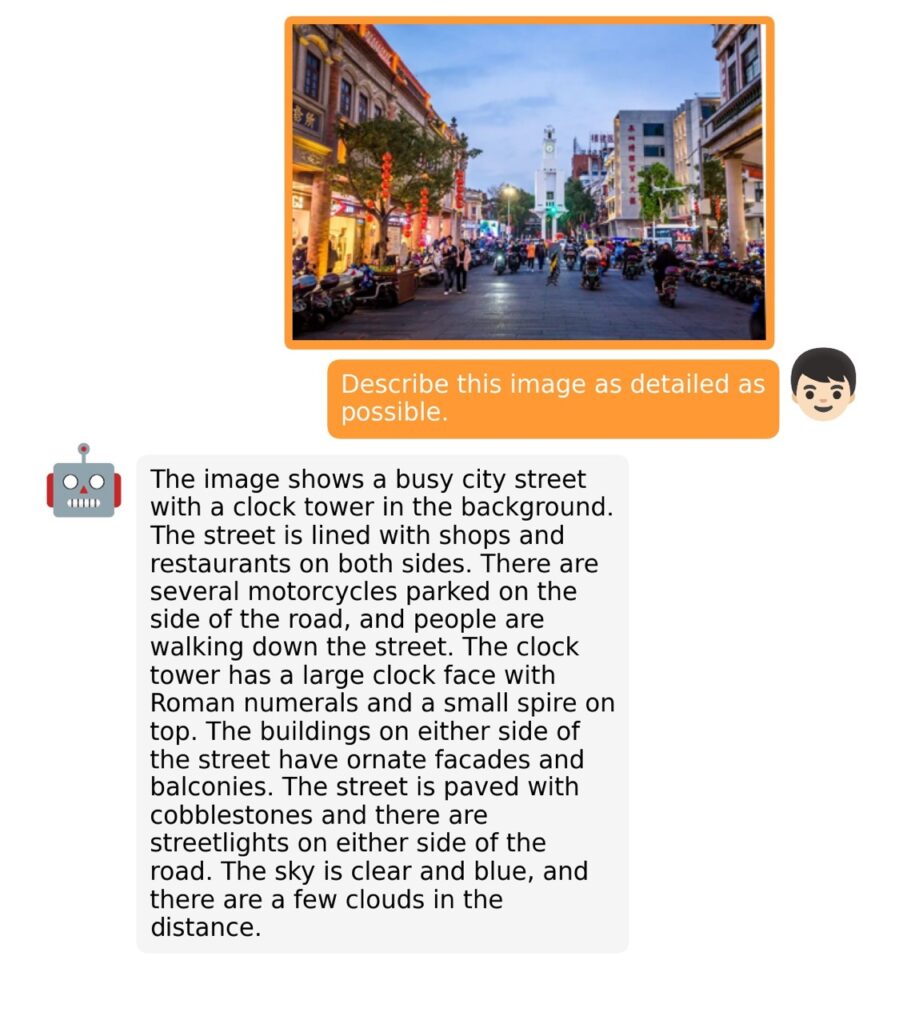
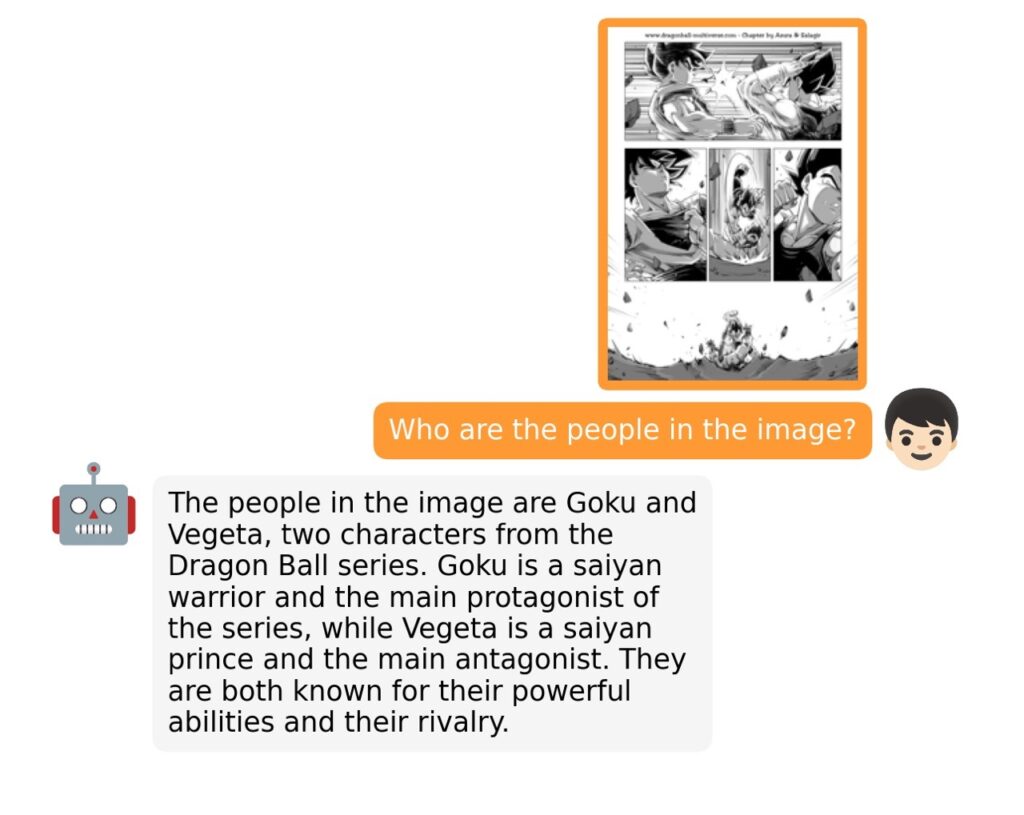
The training process for MiniGPT-4 consists of two stages. The first stage involves pretraining on approximately 5 million aligned image-text pairs. However, this stage can result in unnatural language outputs, including repetition and fragmented sentences. To address this issue and improve usability, researchers propose a novel method to create high-quality image-text pairs using the model itself and ChatGPT. This method results in a small, high-quality dataset used in the second finetuning stage, which significantly improves the model’s generation reliability and overall usability.
Surprisingly, the second stage is computationally efficient, taking only around 7 minutes with a single A100 GPU. MiniGPT-4’s performance highlights the potential of advanced large language models, like Vicuna, for enhancing vision-language understanding in a variety of tasks.