Enhancing Safety and Precision in Autonomous Vehicles through Advanced Stateful Tracking Technology
- Unified Model for Tracking and State Estimation: The newly introduced STT model employs Transformer technology to perform both data association and state estimation simultaneously, enhancing accuracy and reliability in tracking objects for autonomous driving.
- Innovative Metrics for Comprehensive Evaluation: To address the limitations of traditional tracking metrics, STT introduces S-MOTA and MOTPS metrics that evaluate the quality of state estimation alongside data association, providing a more holistic assessment of tracking performance.
- Proven Efficacy on Standard Datasets: Demonstrated on the Waymo Open Dataset, STT showcases competitive real-time performance, affirming its effectiveness in practical autonomous driving scenarios.
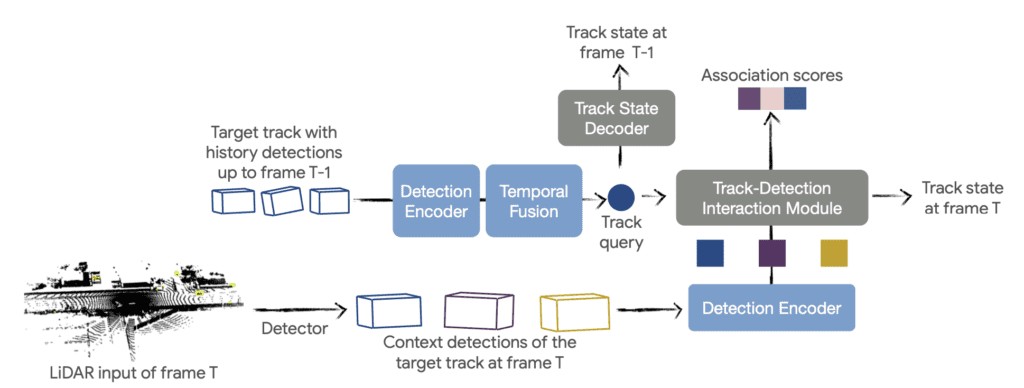
In the realm of autonomous driving, the ability to track objects accurately across multiple frames and predict their future states such as velocity and acceleration is paramount for ensuring safety. Traditional tracking systems often focus on either data association or state estimation, but rarely both effectively, which can compromise the overall performance of autonomous vehicles.
The introduction of STT (Stateful Tracking with Transformers) marks a significant advancement in this field. Developed as a unified framework, STT leverages the power of Transformer architectures to process rich data inputs including appearance, geometry, and motion information over extended detection histories. This integration allows for more accurate and reliable tracking of objects in 3D space, which is critical for navigating complex driving environments.
One of the unique aspects of STT is its revision of traditional evaluation metrics. The model introduces S-MOTA and MOTPS metrics, which extend existing measures to include the quality of state estimation. This is crucial because traditional metrics like MOTA (Multi-Object Tracking Accuracy) and MOTP (Multi-Object Tracking Precision) often fail to account for the precision of state predictions, such as speed and direction, which are critical for making real-time driving decisions.
STT’s performance has been rigorously tested on the Waymo Open Dataset, where it demonstrated strong capabilities in both tracking accuracy and state estimation. These results underscore the model’s potential to significantly enhance the safety and efficiency of autonomous vehicles by providing a more comprehensive understanding of the vehicle’s surroundings.
The development of STT is supported by a notable collaboration among experts in the field, highlighting the collective effort to push the boundaries of what’s possible in autonomous vehicle technology. As autonomous driving continues to evolve, the integration of advanced models like STT will be crucial for developing safer, more reliable autonomous transportation systems. This technology not only represents a step forward in autonomous driving but also sets a new standard for future developments in the field.