Policy Composition Revolutionizes Robotic Learning
- PoCo integrates diverse robotic datasets using generative AI, enhancing multipurpose capabilities.
- MIT’s diffusion-based framework significantly improves task performance and adaptability in robots.
- PoCo addresses the challenge of heterogeneous data, enabling more effective robotic learning and application.
In the ever-evolving field of robotics, one of the most significant challenges has been training general robotic policies from heterogeneous data sources. These sources vary widely, encompassing different modalities such as color, depth, tactile, and proprioceptive information, and are collected across various domains, including simulations, real robots, and human videos. Traditional methods often struggle to manage such diversity, typically focusing on single-domain data to train robots, leading to limitations in versatility and adaptability.
Introducing PoCo: A Unified Approach
MIT researchers have developed a groundbreaking technique called Policy Composition (PoCo), which leverages generative AI models to integrate data from diverse sources, significantly improving the flexibility and efficiency of robotic learning. PoCo allows for the creation of more general robotic policies that can perform a wide range of tasks in various settings, addressing the critical challenge of data heterogeneity.
“Our approach with PoCo is akin to using all the diverse data available, much like how ChatGPT has been trained,” says Lirui Wang, an electrical engineering and computer science graduate student at MIT and lead author of the paper on PoCo. “This is a crucial step for the robotics field.”
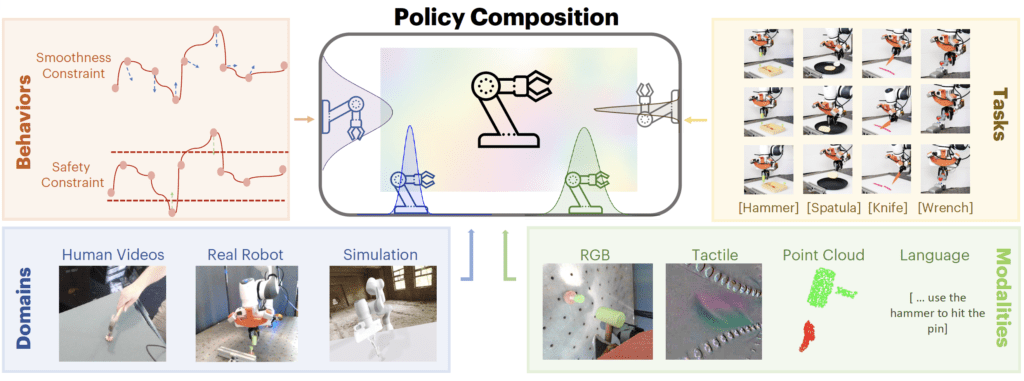
How PoCo Works
PoCo operates by training separate diffusion models on different datasets. Each model learns a specific policy for completing a particular task using one dataset. The individual policies are then combined into a general policy, enabling a robot to perform multiple tasks in various environments.
Diffusion models, typically used for image generation, create new data samples by iteratively refining their outputs. In PoCo’s case, these models are trained to generate trajectories for robots, gradually removing noise from training data to refine their outputs into usable trajectories.
The method, known as Diffusion Policy, builds on previous work by MIT, Columbia University, and the Toyota Research Institute. PoCo enhances this technique by combining policies from different diffusion models, ensuring that the resulting policy meets the objectives of each individual model.
Real-World and Simulation Success
The researchers tested PoCo in both simulations and real-world experiments, focusing on tool-use tasks such as using a hammer or a spatula. The results were impressive, with PoCo leading to a 20 percent improvement in task performance compared to baseline methods.
“One of the benefits of this approach is that we can combine policies to get the best of both worlds. For instance, a policy trained on real-world data might achieve more dexterity, while a policy trained on simulation data might achieve more generalization,” explains Wang. “The composed trajectory looks much better than either one of them individually.”
Future Directions
The team plans to extend PoCo’s capabilities to long-horizon tasks where robots need to use multiple tools sequentially. They also aim to incorporate larger robotics datasets to further enhance performance.
Jim Fan, senior research scientist at NVIDIA and leader of the AI Agents Initiative, praised PoCo’s approach: “We will need all three kinds of data to succeed for robotics: internet data, simulation data, and real robot data. How to combine them effectively will be the million-dollar question. PoCo is a solid step on the right track.”
PoCo represents a significant advancement in robotic learning, offering a flexible, efficient way to train robots using diverse datasets. By integrating data across domains, modalities, and tasks, PoCo not only improves robotic performance but also opens new possibilities for multipurpose robotic applications. This research, funded by Amazon, the Singapore Defense Science and Technology Agency, the U.S. National Science Foundation, and the Toyota Research Institute, is set to revolutionize how we approach robotic learning and deployment.