Bridging the Gap in AI-Generated Imagery with Advanced Image-to-Text Alignment Techniques
- Addressing Misalignment Challenges: CoMat tackles the persistent issue of misalignment between text prompts and generated images in text-to-image diffusion models, attributed to inadequate token attention and insufficient condition utilization.
- Innovative Fine-Tuning Strategy: Introducing an end-to-end diffusion model fine-tuning approach, CoMat integrates an image-to-text concept matching mechanism, leveraging image captioning to enhance text-image alignment without relying on additional datasets.
- Attribute Concentration Module: A novel component of CoMat, the attribute concentration module, is designed to solve the attribute binding problem, further improving the relevance and accuracy of generated images.
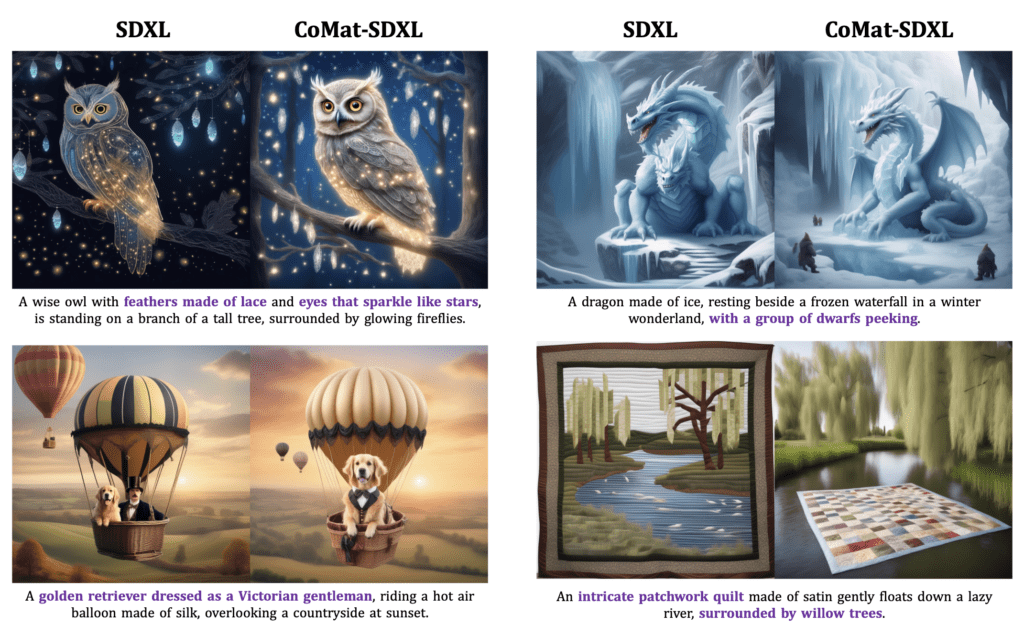
The realm of AI-generated artistry has seen remarkable advancements with the advent of diffusion models, especially in the text-to-image generation domain. Despite the progress, a critical challenge persists: the frequent misalignment between user-input text prompts and the resultant images. This issue often manifests as a deviation from the intended content or attributes described in the text, leading to outputs that, while impressive, may not fully capture the user’s vision.
Enter CoMat, a groundbreaking solution designed to bridge this gap. The core innovation behind CoMat lies in its unique approach to fine-tuning existing diffusion models, specifically by incorporating an image-to-text concept matching mechanism. This mechanism employs an image captioning model to assess the alignment between generated images and the original text prompts, effectively guiding the diffusion model to re-evaluate and adjust its focus on previously overlooked tokens.
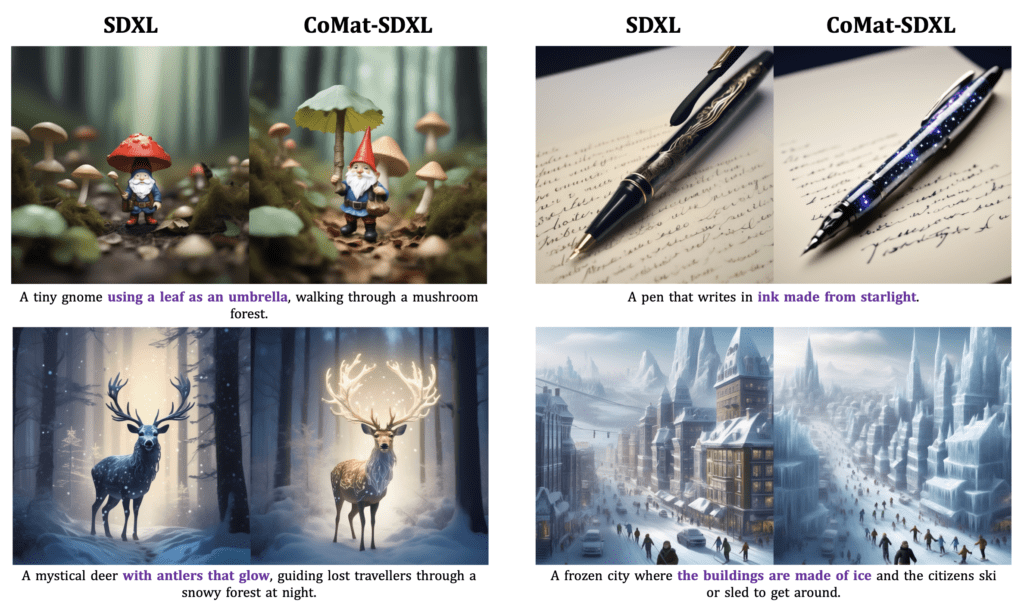
The introduction of CoMat’s attribute concentration module represents a significant leap forward, addressing the complex issue of attribute binding in image generation. This module ensures that attributes described in the text, such as colors, sizes, or other descriptors, are accurately reflected in the corresponding elements of the generated images, enhancing the fidelity and specificity of the output.
One of the most compelling aspects of CoMat is its efficiency and accessibility. By fine-tuning an existing model like SDXL with just 20,000 text prompts, CoMat achieves remarkable improvements in text-to-image alignment, as demonstrated by its superior performance in benchmarks against the baseline SDXL model. This level of enhancement, achieved without the need for extensive image datasets or human preference data, marks a significant advancement in the field.
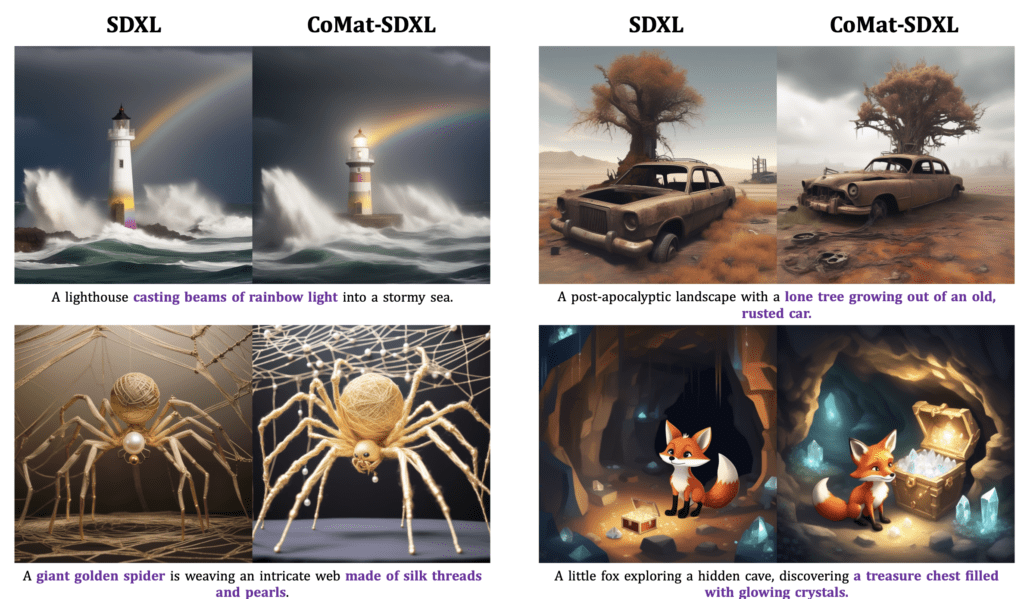
Choosing the right image captioning model is critical for CoMat’s success. The model must exhibit high sensitivity to the nuances of the prompts, including the accurate representation of attributes, relationships, and quantities described in the text. This sensitivity ensures that the concept matching mechanism can effectively differentiate between accurately and inaccurately described images, further refining the alignment process.
CoMat stands not just as a tool but as a beacon for future developments in AI-generated imagery, promising a new era where the visions articulated in text prompts are vividly and accurately brought to life. As the field continues to evolve, CoMat’s innovative approach to addressing text-to-image misalignment through concept matching and attribute concentration is poised to inspire further research and development, pushing the boundaries of what’s possible in AI-powered creativity.